Explainable AI (XAI) plays an essential role in building trust in educational technology by making the processes behind AI decisions clear to users like you. When you understand how these systems arrive at their conclusions, it enhances your confidence in their reliability. XAI provides transparency, helping to address ethical concerns and ensuring fair treatment in learning environments. It also allows you to engage more effectively with educational tools by offering insights into personalized recommendations. As XAI evolves, you'll find even more ways it empowers both students and educators, deepening trust and collaboration in the learning experience.
Key Takeaways
- Explainable AI (XAI) enhances user understanding by providing clear, human-readable explanations of AI decisions in educational tools.
- Transparency in AI decision-making fosters trust and boosts user engagement with educational technology.
- XAI promotes fairness by reducing biases in assessments and ensuring equitable access to educational resources.
- Clear rationales from XAI facilitate informed decision-making, empowering users to navigate educational tools effectively.
- Trust in AI-driven systems is strengthened through accountability, compliance with regulations, and ethical practices fostered by XAI.
Understanding Explainable AI
In today's educational landscape, understanding Explainable AI (XAI) is more important than ever. XAI clarifies AI decision-making processes, offering clear, human-understandable explanations for AI outcomes. This focus on transparency is vital for building trust among educators and learners who rely on educational technology.
When you incorporate XAI into your tools, you enable users to grasp how recommendations are made, which enhances their confidence in the system. Additionally, as AI security integrates existing systems, it brings an added layer of protection to educational technologies, guaranteeing that user data remains confidential and secure.
Techniques like LIME and SHAP help demystify complex AI models. These methods guarantee that even non-expert users can interpret the results, making educational technology more accessible. When stakeholders understand the reasoning behind AI decisions, they can better engage with the technology and feel empowered in their learning journeys.
Moreover, XAI addresses ethical AI concerns, emphasizing fairness, accountability, and transparency (FATE). By prioritizing these principles, XAI creates equitable learning environments, where every user feels valued and understood.
Ultimately, when you embrace Explainable AI, you foster trust, paving the way for successful adoption of AI technologies in educational settings. Understanding XAI isn't just beneficial; it's vital for the future of education.
Importance of Transparency
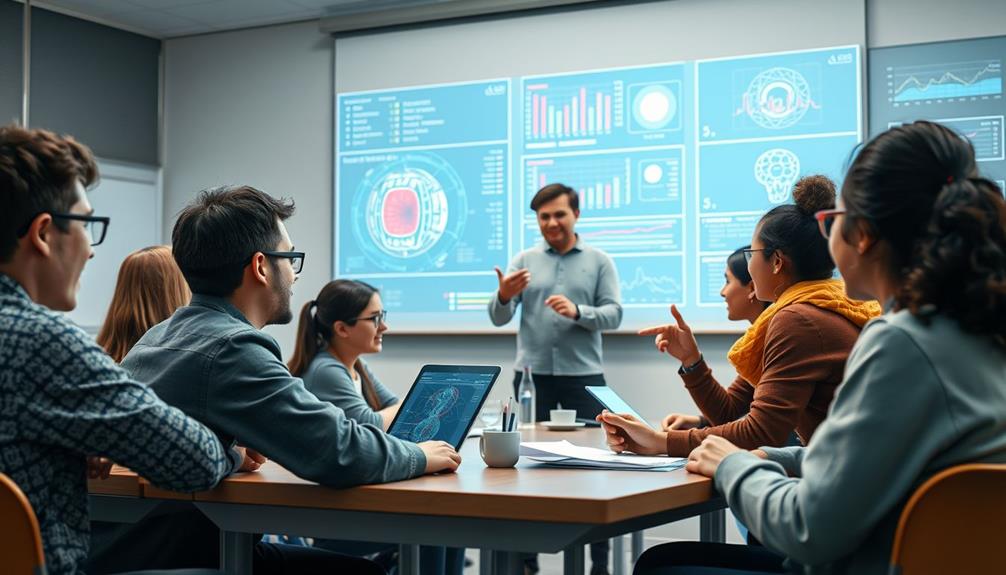
Transparency in educational technology is essential for helping you understand how AI systems make decisions.
This clarity is similar to how metrics track visitor engagement, providing insights that enhance user experience.
When you can see the reasoning behind recommendations, it not only boosts your confidence in the tools but also encourages ethical practices.
Enhancing User Understanding
Often, users find it challenging to trust AI systems when they don't understand how decisions are made. This is where Explainable AI (XAI) plays a crucial role in enhancing user understanding within educational technology. By offering clear and interpretable explanations for AI decisions, XAI fosters transparency, demystifying how these systems operate.
As AI technologies continue to evolve and impact various sectors, including education, it becomes important to prioritize ethical considerations and user privacy in their deployment ethical frameworks required.
Here are three key benefits that come from this transparency:
- Increased Engagement: When users receive understandable AI-generated feedback and rationales, their satisfaction and engagement with educational tools drastically increase.
- Effective Utilization: Visualization methods and interactive interfaces help you grasp the reasoning behind AI outputs, making it easier to use educational technologies effectively.
- Trust Building: As transparency improves, you'll find it easier to trust the AI systems, leading to broader adoption and acceptance among students, educators, and administrators.
Incorporating Explainable AI in educational technology not only enhances user understanding but also aligns with ethical guidelines and regulatory standards.
Ultimately, this approach guarantees that AI systems promote fair and equitable learning environments, making education more accessible and effective for everyone involved.
Promoting Ethical Practices
Trust is fundamental in fostering ethical practices within educational technology, especially when it comes to AI systems. Explainable AI (XAI) enhances transparency by providing clear rationales for AI-driven decisions, which helps you, as an educator or learner, hold the system accountable.
When you understand how AI recommendations are made, it builds trust and reduces skepticism towards automated tools. Additionally, understanding the nuances of cybersecurity challenges in educational technology is essential for safeguarding sensitive data and maintaining user confidence.
Transparency in AI algorithms is vital for identifying biases and ensuring equitable access to educational resources. By integrating XAI, you can actively participate in discussions about fairness and ethics in educational technology. This understanding allows you to recognize potential biases that could affect learning outcomes and advocate for more equitable practices.
Moreover, XAI supports compliance with privacy regulations like GDPR by making data usage and decision-making processes clear. When you know how your data is used, it fosters a sense of security and trust in the system.
Ultimately, implementing XAI not only enhances user satisfaction but also empowers you as a learner, making you feel more engaged when you grasp the reasoning behind AI-supported interventions.
Benefits of XAI in Education
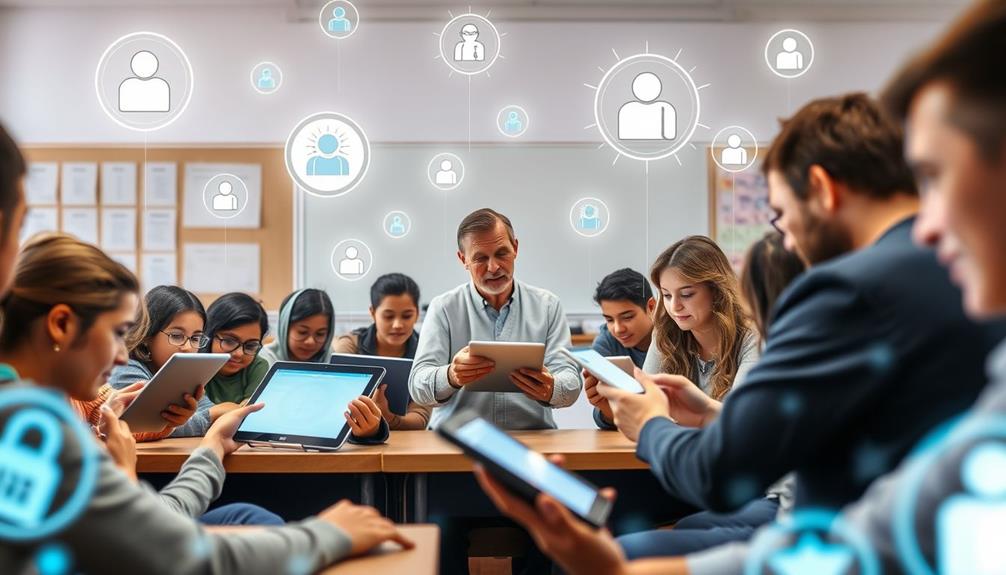
When you incorporate Explainable AI in education, you enhance transparency, allowing both educators and students to grasp the reasoning behind AI-driven decisions.
This clarity not only promotes fairness and equity but also supports informed decision-making, ensuring all students receive fair treatment.
Additionally, as certain fields remain resilient against AI disruption, the integration of XAI can foster a more secure learning environment.
As you explore these benefits, you'll see how XAI can transform the educational experience for everyone involved.
Enhancing Transparency in Education
As educators increasingly integrate technology into the classroom, explainable AI (XAI) emerges as an essential tool for enhancing transparency in education. By providing clear rationales for AI-driven decisions, XAI helps you and your students understand the reasoning behind recommendations and assessments.
This transparency fosters a deeper trust in the educational tools you use, similar to how effective communication strategies enhance caregiver-patient relationships in caregiving.
Here are three key benefits of XAI in education:
- Improved Trust: Users are more likely to engage with systems that transparently communicate their processes and outcomes, leading to increased confidence in the technology.
- Personalized Learning Paths: Utilizing XAI methods like open learner models allows you to present personalized learning paths that are easily interpretable, fostering collaboration and understanding.
- Ethical Practices: XAI supports compliance with educational data regulations, ensuring AI systems remain transparent and accountable, which is vital for ethical practices in handling student data.
Promoting Fairness and Equity
Explainable AI (XAI) helps level the playing field in education by promoting fairness and equity through transparency. When you use XAI, you get clear rationales for AI-driven decisions, allowing both educators and students to understand how recommendations are made. This understanding is essential in reducing biases in grading and assessment.
Additionally, just like how unique traits of English Cream Dachshunds can help owners understand their pets better, XAI provides insights that help educators and students navigate educational tools effectively.
With XAI, everyone involved—students, teachers, and administrators—can access comprehensible explanations of AI outputs. This access guarantees equity, especially for those from diverse backgrounds. XAI tools can identify and mitigate potential biases within algorithms, tailoring support to meet each student's individual needs and learning styles.
Furthermore, XAI fosters trust in educational technology by increasing transparency. When educators know they can verify and validate AI decisions, they're more likely to adopt these tools. This environment encourages you, as a student, to engage actively with educational interventions.
Supporting Informed Decision-Making
Transparency in educational technology empowers informed decision-making by providing clear rationales for AI-driven interventions.
Just as understanding the ingredients in food can help individuals make better dietary choices, when you understand the reasons behind personalized learning recommendations, you can make better choices that positively impact your students.
Explainable AI (XAI) enhances this transparency, helping you identify potential biases in AI systems and ensuring equitable access to resources, much like how vegan status of common foods informs dietary decisions.
Here are three key benefits of XAI in supporting informed decision-making:
- Enhanced Understanding: XAI clarifies how AI models arrive at their conclusions, enabling you to grasp the logic behind decisions.
- Bias Detection: With transparent insights, you can spot and address biases in AI systems, promoting fairness in student assessments and interventions.
- Collaboration: XAI fosters trust among stakeholders—teachers, students, and parents—by making AI decision-making processes more understandable, encouraging collective input and feedback.
Real-World Applications of XAI
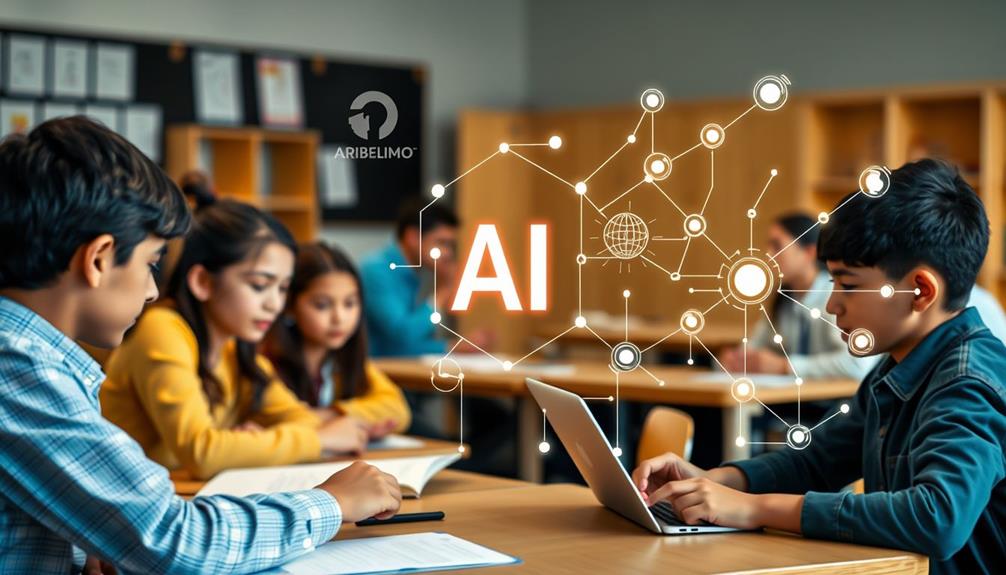
In today's educational landscape, the integration of explainable AI (XAI) is revolutionizing how students and teachers interact with learning tools. Explainable AI systems provide transparent feedback on student performance, allowing you, as a teacher, to understand the reasoning behind assessments and adapt your instructional strategies accordingly. This level of trust and transparency fosters a more collaborative learning environment.
Additionally, similar to how AI influences composition in music, XAI tools enable educators to compose personalized learning experiences based on individual student data.
XAI tools, like open learner models, facilitate personalized learning experiences by explaining the basis for AI-driven recommendations tailored to individual student needs. This helps you guide your students effectively, ensuring they receive the support necessary for their unique learning patterns.
Moreover, the use of XAI in educational interventions actively identifies and mitigates biases in grading systems, promoting fair evaluations across diverse populations. Real-world case studies highlight how XAI enhances student engagement and motivation, clarifying how AI-derived suggestions can lead to improved learning outcomes.
Additionally, XAI's real-time explanations within adaptive learning platforms empower students to understand their learning journeys better, encouraging self-regulation and a growth mindset. Embracing XAI in educational technology not only elevates teaching practices but also strengthens the overall learning experience.
Challenges of Implementing XAI
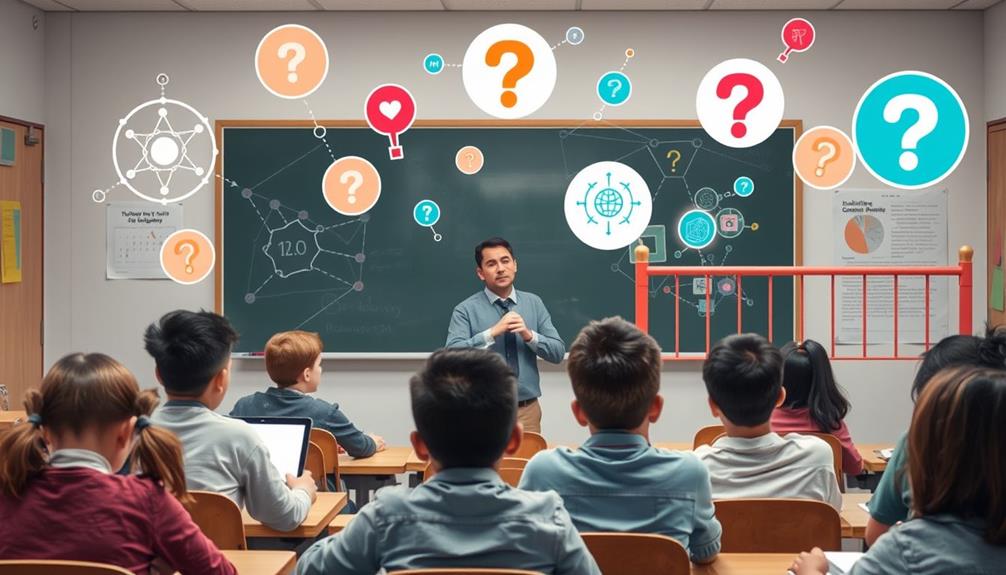
When you look at implementing XAI in educational technology, you'll quickly realize that the complexity of AI models can make it tough to provide clear explanations.
This complexity often results in varying interpretations from users, which can create confusion and skepticism.
Additionally, the importance of social proof in building trust can be a significant factor, as positive feedback from educators can enhance user confidence in these technologies.
Addressing these challenges is essential for fostering trust and ensuring effective use of AI in educational settings.
Complexity of AI Models
Traversing the complexity of AI models can be intimidating, especially in educational technology where understanding decision-making processes is fundamental.
The intricate nature of these models often means they function as "black boxes," leaving you puzzled about how decisions are made. This lack of transparency can undermine trust in AI systems, making it imperative to find ways to enhance explainability without sacrificing performance.
Here are three key challenges you might face when dealing with the complexity of AI models:
- Trade-offs: Simplifying AI models to improve interpretability often comes at the cost of accuracy and effectiveness.
- User Variation: Different users, with diverse backgrounds and expertise, may interpret AI outputs in various ways, complicating the delivery of clear explanations.
- Ethical Concerns: Misunderstandings can lead to biases and unfair outcomes, highlighting the need for ethical implementation of AI in educational contexts.
Addressing these challenges is essential for fostering trust and ensuring that AI tools are both effective and fair in their application.
Ongoing innovation in explainable AI (XAI) techniques is crucial for bridging the gap between model complexity and user comprehension.
User Interpretation Variability
User interpretation variability poses significant challenges in implementing explainable AI (XAI) in educational technology. When users have different backgrounds and levels of expertise, their understanding of AI explanations can greatly differ. This variability often leads to misinterpretations, which can undermine trust in these AI tools.
If you're not familiar with AI concepts, you might find the explanations overly complex or unclear, making it hard to see how they apply to your learning. The intricacies of deep learning models can exacerbate these issues, resulting in AI explanations that aren't easily digestible for everyone.
To effectively implement XAI, it's essential to develop tailored communication strategies that cater to diverse audiences. By ensuring that explanations are accessible, you can enhance user comprehension and, ultimately, trust in educational technology.
Ongoing feedback mechanisms and user testing play an important role in identifying interpretation challenges. By actively seeking input from users, developers can refine AI explanations, making them clearer and more relevant.
This iterative process not only improves understanding but also builds confidence in the technology, fostering a more supportive learning environment.
Future Trends in Explainable AI
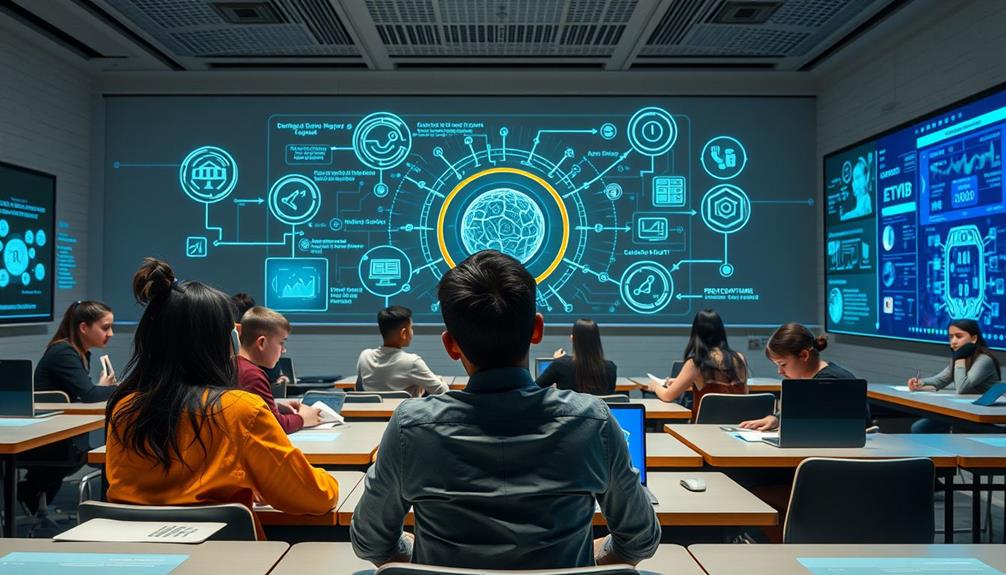
As we look ahead to the future of Explainable AI (XAI) in educational technology, a clear trend emerges: the development of user-friendly interfaces designed to improve transparency and comprehension for both educators and learners.
This evolution will greatly enhance trust in AI-driven decisions, allowing you to better understand how these technologies impact learning.
Here are three key trends to watch for:
- Improved Interpretability: Techniques like Layer-wise Relevance Propagation and SHAP will offer clearer insights into AI recommendations, making it easier for you to trust the feedback you receive.
- Regulatory Compliance: With frameworks like the EU's AI Act, educational technologies will need to meet regulatory requirements, ensuring ethical standards and accountability in AI applications.
- Customized Explanations: Ongoing research will focus on tailoring explanations to accommodate diverse user backgrounds, ensuring that everyone can access and understand AI decision-making processes.
Incorporating these trends will foster transparency and trust, paving the way for more effective educational tools that you can confidently use in your teaching and learning experiences.
Building Trust Through XAI
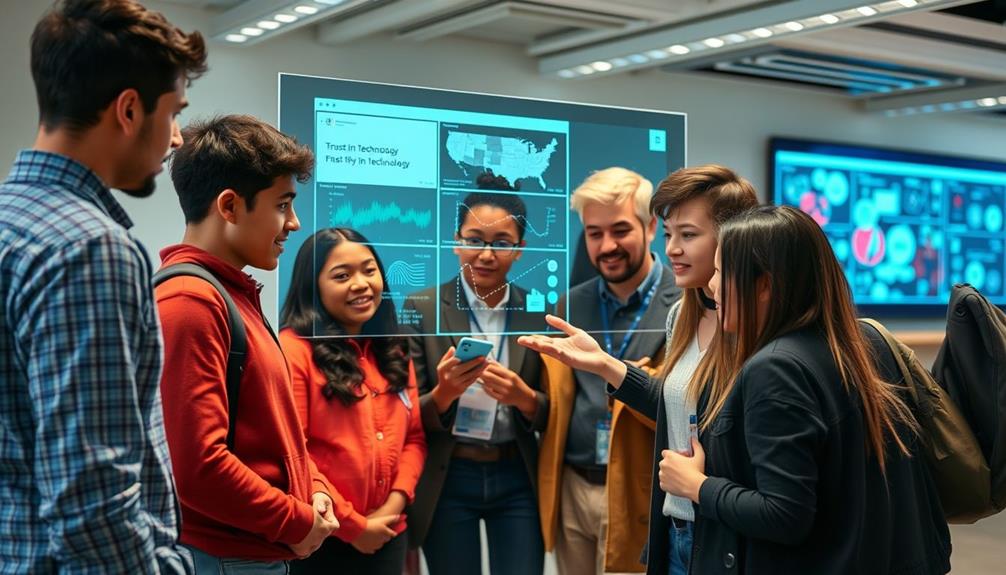
The growing emphasis on user-friendly interfaces in Explainable AI is just the beginning of a larger movement toward building trust in educational technology. By providing clear, understandable explanations for AI-driven decisions, Explainable AI enhances your ability to assess the reliability of educational interventions. Tools like LIME and SHAP personalize insights, showing how individual student data influences learning outcomes, which helps you feel more confident in the technology.
Moreover, the implementation of XAI addresses significant concerns about fairness and bias. This guarantees equitable access to learning resources, promoting a just educational experience for all students. As educational institutions increasingly adopt Explainable AI, they meet transparency requirements and regulatory compliance standards, reinforcing your trust in AI applications.
Building trust through XAI goes beyond just technology; it involves ongoing communication and engagement with educators and learners. By making sure you understand the limitations and capabilities of AI tools, educational technology becomes a more reliable partner in your learning journey.
In this way, Explainable AI not only enhances trust but also fosters a collaborative environment where everyone can thrive.
Framework for Educational AI Tools
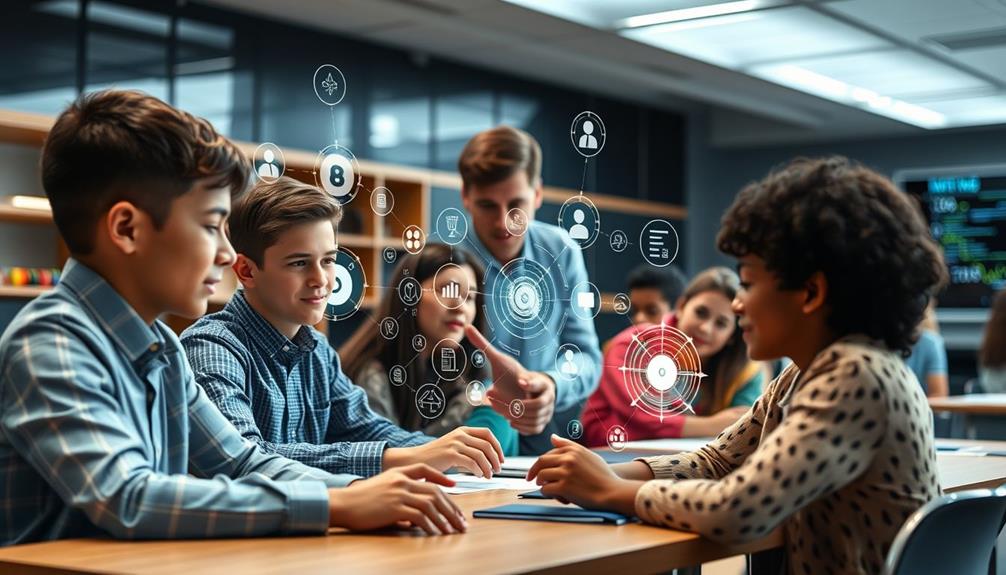
Creating a robust framework for educational AI tools hinges on understanding the diverse needs of all stakeholders involved. By prioritizing explainability, you can foster user trust and guarantee that AI models serve their intended purpose in educational settings.
Here are three key aspects to reflect upon:
- Stakeholder Involvement: Engage educators, students, and administrators in the design process to guarantee the tools meet their needs and expectations.
- Explanation Presentation: Use user-friendly interfaces that present explanations in accessible formats, like visual aids and interactive elements, so everyone can understand the AI's reasoning.
- Human-Centered Design: Focus on creating tools that not only deliver insights but also enhance the learning experience through intuitive designs that cater to diverse audiences.
Case Studies in XAI
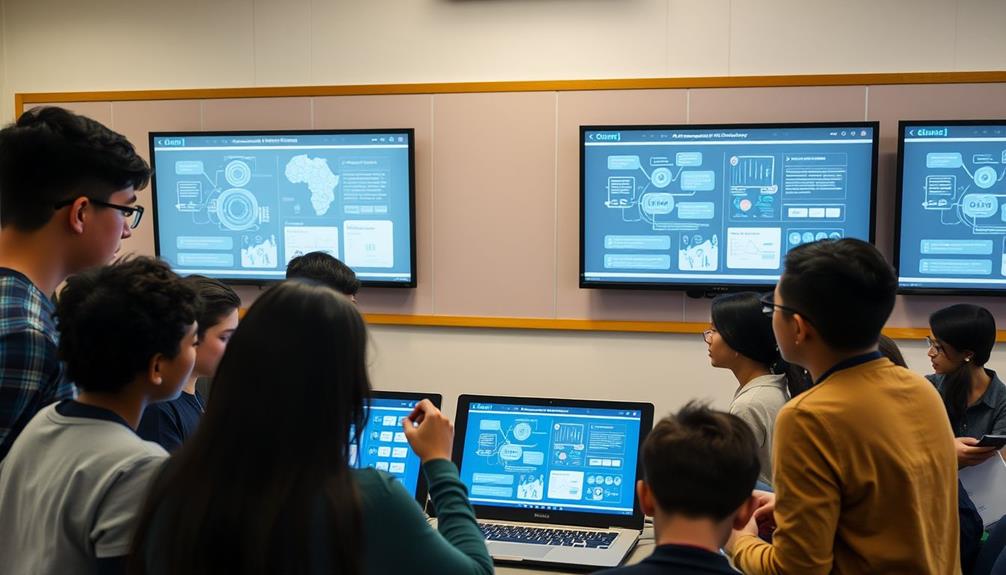
In exploring the impact of explainable AI (XAI) in educational technology, you'll find compelling case studies that illustrate how these models can greatly boost student engagement and comprehension.
One notable case study involved open learner models, which transparently communicate students' progress and areas for improvement. This transparency fosters greater learner autonomy, enhancing overall trust in the system.
Another research project implemented XAI in adaptive learning systems, leading to a 20% increase in student satisfaction. Students gained clearer insights into their personalized learning paths, which encouraged them to take ownership of their learning.
Additionally, a tutoring system used SHAP to explain its recommendations, resulting in a remarkable 30% improvement in student performance on assessments. By clarifying the rationale behind suggested learning activities, the system built trust and encouraged active participation.
A thorough framework for XAI in education emerged from these case studies, emphasizing the importance of stakeholder involvement and the need for clear, accessible explanations tailored to diverse audiences.
These examples underscore how explainable AI can enhance student engagement and foster a deeper understanding of learning processes in educational technology.
Opportunities for Future Research
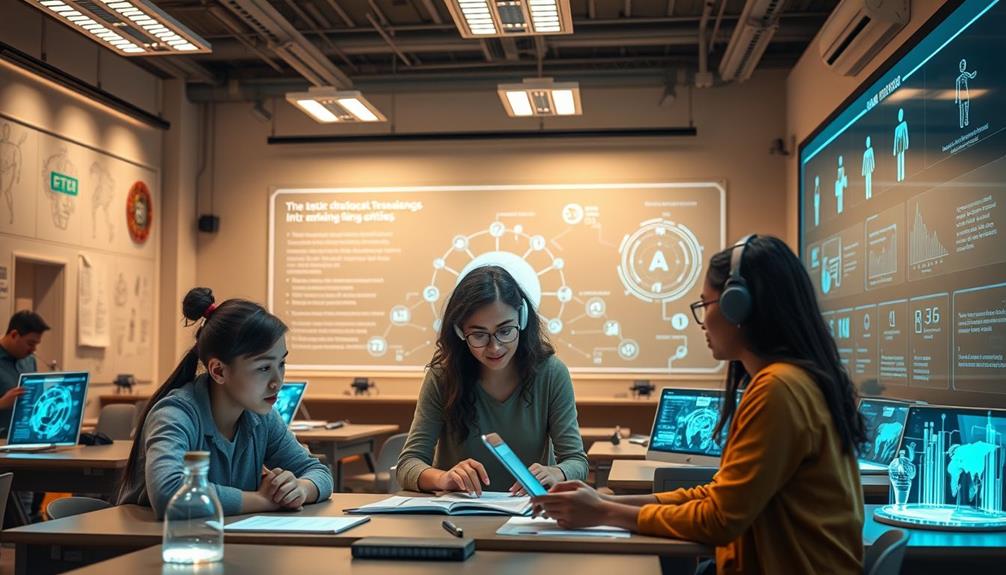
Building on the insights gained from case studies in explainable AI (XAI), several promising avenues for future research can be explored. You can immerse yourself in how XAI can enhance educational technology by focusing on frameworks that balance explainability and effectiveness.
Understanding the impact of XAI on student engagement and learning outcomes will also be essential in demonstrating its value in educational settings.
Here are three key opportunities for future research:
- Stakeholder Needs: Investigate the diverse needs of students, educators, and administrators to develop tailored explainable AI solutions that cater to different user profiles.
- Integration Techniques: Explore how XAI techniques can be integrated with existing educational technologies to promote transparency and build trust among users in real-world classroom environments.
- Ethical Concerns: Examine the ethical implications of bias and fairness in educational AI systems, focusing on XAI's role in ensuring equitable educational interventions.
Frequently Asked Questions
How Does Explainability Contribute to Trust in Ai?
When AI systems offer clear explanations for their decisions, you feel more confident in their reliability. This transparency helps you understand how outcomes are reached, fostering trust and encouraging you to engage more with the technology.
What Is the Significance of Explainability of Trusted AI Systems?
Understanding the significance of explainability in trusted AI systems means recognizing how clear, transparent reasoning boosts user confidence. When you see how decisions are made, you're more likely to engage and trust the technology.
What Is the Significance of Explainability of Trusted AI Systems?
Explainability in trusted AI systems matters because it helps you understand decision-making processes. When you see clear rationales behind outputs, you're more likely to trust the technology, leading to better engagement and informed decisions.
What Is the Role of Explainable Ai?
Imagine traversing a maze without a map—you'd feel lost. Explainable AI helps you understand complex decisions, clarifying how outcomes are reached. It empowers you to trust the process, enhancing your confidence in using AI effectively.
Conclusion
In the rapidly evolving world of educational technology, explainable AI isn't just a buzzword; it's the bridge to trust. By embracing transparency and understanding, you can empower both educators and learners to engage confidently with AI tools. As you advocate for clearer insights and ethical practices, remember: trust isn't built overnight, but with every step toward clarity, you're paving the path for a brighter, more inclusive educational future. Together, let's shape a landscape where everyone thrives.