AI Security
Exploring the Human Impact of Ethical AI Security
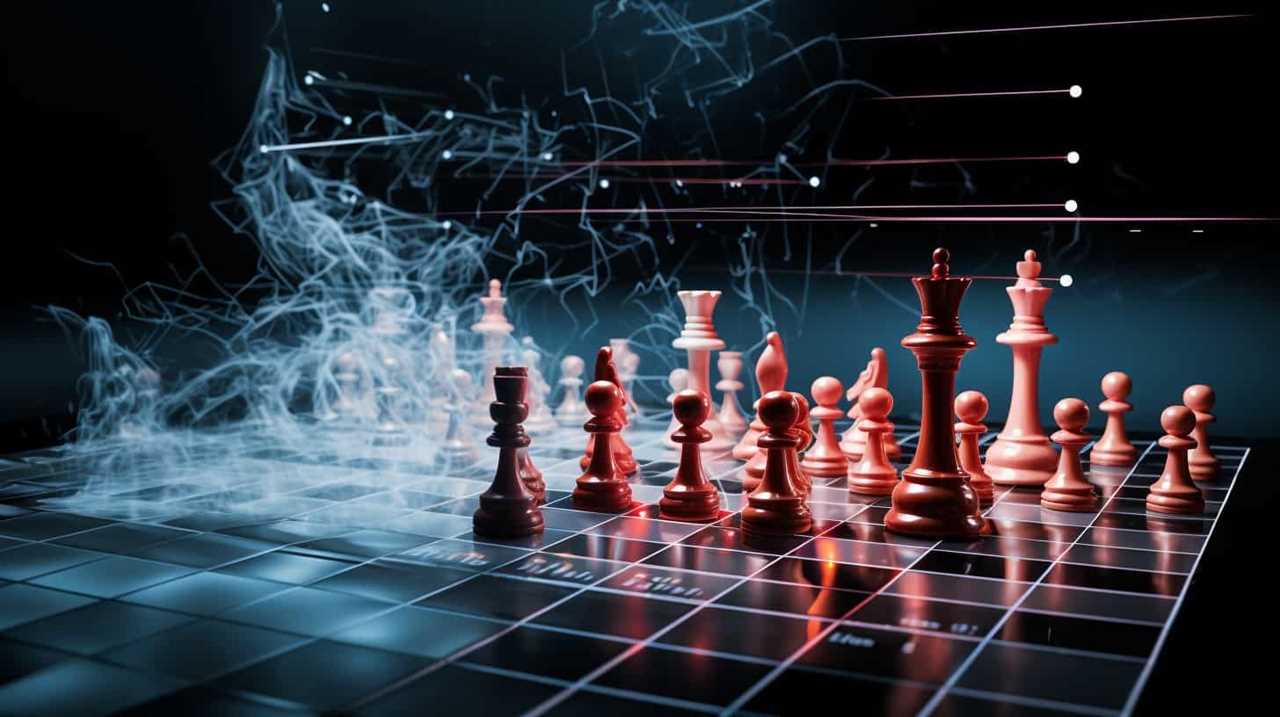
We explore the complex world of ethical AI security and how it deeply affects humanity.
As technology continues to advance, the need to address the human implications of AI security becomes increasingly pressing.
In this article, we uncover the potential consequences of privacy concerns, bias, and ethical challenges in AI decision-making.
We explore the importance of transparency, accountability, and the cultural implications surrounding AI security.
Join us on this journey as we navigate the complex web of ethical AI security and its far-reaching effects.
Key Takeaways
- Data ownership and control are critical considerations in AI systems.
- Balancing security and privacy is essential for ethical AI use.
- Bias and discrimination in AI systems need to be addressed through techniques like bias detection and algorithmic auditing.
- Transparency, accountability, and human oversight play important roles in ethical AI decision-making.
Privacy Concerns in AI Security
Privacy concerns arise when utilizing artificial intelligence (AI) security measures. As AI systems become more prevalent in our lives, the issue of data ownership becomes paramount. With the vast amount of data being collected and analyzed, it’s essential to address who’s control and access to this data.
Furthermore, the increasing use of AI for surveillance purposes raises significant risks. The potential for misuse and abuse of surveillance technologies is a valid concern, as it can infringe upon individuals’ privacy and civil liberties. Striking a balance between security and privacy is crucial to ensure the ethical use of AI systems.
As we delve into the subsequent section about ‘bias and discrimination in AI systems,’ it’s important to acknowledge that privacy concerns are just one aspect of the broader ethical implications of AI security.
Bias and Discrimination in AI Systems
When exploring the human impact of ethical AI security, it’s important to address the issue of bias and discrimination in AI systems.
Bias detection and algorithmic auditing are crucial in ensuring that AI systems are fair and unbiased. Bias in AI systems can arise from various sources, such as biased training data, biased algorithms, or biased decision-making processes.
To address this, bias detection techniques can be employed to identify and measure the presence of bias in AI systems. Algorithmic auditing involves analyzing the algorithms and decision-making processes to uncover any biases that may exist.
By implementing robust bias detection and algorithmic auditing practices, we can mitigate the potential harm caused by biased AI systems and strive towards fair and inclusive AI technologies.
Now, let’s delve into the ethical challenges in AI decision-making.
Ethical Challenges in AI Decision-Making
As we delve into the ethical challenges in AI decision-making, it’s imperative to consider the potential risks associated with biased algorithms and the need for comprehensive safeguards. Ethical considerations play a crucial role in ensuring that AI systems make fair and unbiased decisions.
Here are four key ethical challenges that arise in AI decision-making processes:
- Fairness: Ensuring that AI algorithms don’t discriminate against individuals based on attributes such as race, gender, or socioeconomic status.
- Accountability: Holding AI systems accountable for their decisions by establishing clear lines of responsibility and liability.
- Transparency: Making AI algorithms transparent and understandable to users and stakeholders, allowing for scrutiny and preventing the ‘black box’ problem.
- Human oversight: Ensuring that humans have the ability to intervene and override AI decisions when necessary, to prevent potential harm or ethical violations.
These ethical considerations are crucial in guiding the development and deployment of AI systems that align with our values and promote fairness and justice.
Transitioning into the subsequent section about ‘transparency and accountability in AI algorithms’, we’ll further explore how these principles can be implemented to address the challenges discussed.
Transparency and Accountability in AI Algorithms
When it comes to transparency and accountability in AI algorithms, one of the primary concerns is ensuring algorithmic fairness. This means that the algorithms shouldn’t exhibit biases or discrimination against certain individuals or groups.
Achieving algorithmic fairness requires a thorough understanding of the data used to train the algorithms and continuously monitoring and evaluating their outcomes.
Beyond fairness, there are also legal and ethical implications associated with AI algorithms, such as privacy, consent, and responsibility for the decisions made by these algorithms.
Ensuring Algorithmic Fairness
We prioritize the incorporation of transparency and accountability into AI algorithms to ensure algorithmic fairness. This is crucial in addressing the issue of algorithmic bias, where AI systems may unintentionally discriminate against certain individuals or groups.
To achieve algorithmic fairness, we advocate for the implementation of explainable AI, which allows users to understand the reasoning behind AI decisions. This transparency helps identify any biases or unfairness in the algorithms and enables appropriate corrective actions to be taken.
Additionally, we emphasize the importance of continuously monitoring and evaluating AI algorithms to ensure they remain fair and unbiased. By promoting transparency, accountability, and ongoing evaluation, we aim to foster trust in AI systems and mitigate the potential negative impacts on individuals and society.
Legal and Ethical Implications
To ensure transparency and accountability in AI algorithms, our approach involves incorporating legal and ethical considerations into the development and deployment of these systems. This includes addressing issues of data ownership and algorithmic bias, which have significant implications for the fairness and reliability of AI technologies.
Data ownership is a critical aspect of AI security and ethics. It raises questions about who has the rights to the data used to train and operate AI algorithms. Ensuring clear ownership and control over data is essential to protect privacy and prevent misuse. Additionally, algorithmic bias is a concern that must be addressed. Biased algorithms can perpetuate discrimination and inequality, leading to unfair outcomes for certain individuals or groups. By implementing measures to detect and mitigate bias, we can enhance the ethical integrity of AI systems.
Data Ownership | Algorithmic Bias |
---|---|
Clear ownership and control over data | Detection and mitigation of bias |
Protection of privacy and prevention of misuse | Fair outcomes for individuals and groups |
Ensuring ethical integrity of AI systems | Addressing discrimination and inequality |
Considering these legal and ethical implications is crucial for the responsible development and deployment of AI algorithms. By incorporating transparency and accountability measures, we can foster trust in AI technologies and ensure that they are used in a fair and unbiased manner.
This focus on transparency and accountability in AI algorithms also has implications for the impact of AI security on job displacement, which we will explore in the next section.
Impact of AI Security on Job Displacement
With the increasing implementation of ethical AI security, concerns arise regarding the potential for job displacement due to automation. As we delve into the impact of AI security on job displacement, it’s crucial to consider the following key points:
- Job creation: While AI may automate certain tasks, it also has the potential to create new job opportunities. As technology advances, new roles emerge that require human skills such as creativity, critical thinking, and emotional intelligence.
- Workforce adaptation: The workforce needs to adapt to the changing landscape by acquiring new skills and knowledge. Lifelong learning and upskilling become essential for individuals to remain competitive in the job market.
- Redistribution of tasks: Rather than completely replacing jobs, AI can augment human capabilities by automating mundane and repetitive tasks. This redistribution of tasks allows humans to focus on higher-value and complex responsibilities.
- Ethical considerations: As AI takes on more tasks, ethical concerns arise. It becomes crucial to ensure that AI systems are designed and implemented ethically, taking into account fairness, transparency, and accountability to mitigate any negative impact on jobs.
Understanding the impact of AI security on job displacement requires a holistic view that considers both the potential risks and opportunities it presents. By proactively addressing the challenges, we can harness the benefits of AI while minimizing the negative consequences.
Trust and User Adoption of AI Technology
One important factor to consider when exploring the human impact of ethical AI security is the level of trust and user adoption of AI technology. User trust is crucial for the successful implementation and acceptance of AI systems. Without trust, users may hesitate to adopt AI technology, fearing ethical implications or potential negative consequences. To better understand the current state of user trust and adoption, let’s examine the following table:
Factors Influencing User Trust | Factors Influencing User Adoption |
---|---|
Transparency | Ease of Use |
Explainability | Performance |
Privacy and Data Security | Compatibility |
Transparency and explainability are key ethical considerations that influence user trust. Users want to understand how AI systems make decisions and the reasoning behind them. Privacy and data security are also important, as users need assurance that their personal information is protected. On the other hand, ease of use, performance, and compatibility are factors that drive user adoption. AI systems must be user-friendly, deliver reliable results, and seamlessly integrate into existing workflows. By addressing these factors, we can build trust and encourage widespread adoption of ethical AI technology.
Cultural Implications of Ethical AI Security
When considering the cultural implications of ethical AI security, we must examine the impact it has on societal norms and values. The cultural adaptation and social acceptance of AI security measures play a vital role in shaping the way individuals and communities perceive and interact with these technologies. Here are four key points to consider:
- Cultural adaptation: Different cultures have varying attitudes towards privacy, surveillance, and technology. Ethical AI security must be developed with cultural sensitivities in mind to ensure it aligns with societal expectations and values.
- Social acceptance: The acceptance of AI security measures within a community is crucial for their effective implementation. Public education and awareness campaigns can help address concerns and build trust, promoting widespread social acceptance.
- Impact on interpersonal relationships: AI security measures may affect the way people relate to one another. For example, increased surveillance can impact trust and privacy within communities, leading to changes in social dynamics and interactions.
- Influence on societal norms: Ethical AI security has the potential to shape and redefine societal norms. It can challenge existing notions of privacy, security, and accountability, prompting discussions and debates about the balance between individual rights and collective safety.
Considering these cultural implications is essential to ensure that ethical AI security measures aren’t only effective but also aligned with societal expectations and values.
Transitioning into the subsequent section about legal and regulatory considerations for AI security, it’s crucial to understand how cultural factors can influence the development and implementation of legal frameworks and regulations in this domain.
Legal and Regulatory Considerations for AI Security
When considering the legal and regulatory aspects of AI security, two key points that require attention are privacy and data protection, as well as liability for AI breaches.
Privacy and data protection are essential to ensure that individuals’ personal information is safeguarded when AI systems are deployed.
Additionally, liability for AI breaches raises important questions about who should be held responsible when AI systems fail to perform as intended.
These considerations highlight the need for robust legal frameworks and regulations to address the unique challenges posed by AI security.
Privacy and Data Protection
Our team is examining the legal and regulatory considerations for privacy and data protection in the context of AI security. In order to fully understand the implications of AI security on privacy and data protection, it’s important to consider the following:
- Data Governance: Establishing robust data governance policies and practices is crucial to ensure the responsible and ethical use of data in AI systems. This includes defining data ownership, access controls, and data classification frameworks.
- User Consent: Obtaining informed and explicit user consent is paramount when collecting and processing personal data for AI security purposes. Organizations must ensure that individuals are fully aware of how their data will be used and have the right to withdraw consent at any time.
- Legal Frameworks: Adhering to existing privacy and data protection laws, such as the General Data Protection Regulation (GDPR), is essential when implementing AI security measures. Organizations must comply with these regulations to safeguard individuals’ personal information.
- Accountability and Transparency: It’s crucial that organizations are transparent about their data processing practices and are accountable for any breaches or misuse of personal data. This includes conducting regular audits, implementing security measures, and providing individuals with mechanisms to exercise their rights.
Liability for AI Breaches
What legal and regulatory considerations must be taken into account regarding liability for AI breaches in the context of AI security?
When it comes to AI security, it’s essential to establish a liability framework that holds individuals and organizations responsible for any breaches that may occur. The rapidly evolving nature of AI technology presents unique challenges for determining liability, as traditional legal frameworks may not adequately address the complexities involved. Therefore, it’s crucial for policymakers and regulatory bodies to develop comprehensive guidelines and frameworks that address the potential risks and consequences associated with AI breaches.
Additionally, corporate responsibility plays a significant role in ensuring accountability and minimizing the human impact of AI breaches. Organizations must prioritize the development and implementation of robust security measures and proactive risk management strategies to mitigate the potential harm caused by AI breaches. By doing so, they demonstrate their commitment to ethical AI practices and protect individuals from the adverse effects of AI security breaches.
Frequently Asked Questions
How Can Individuals Protect Their Privacy When Using Ai-Powered Devices or Services?
To protect our privacy when using AI-powered devices or services, we must take steps to safeguard our data and address privacy concerns. This can involve managing permissions, using strong encryption, and being mindful of the information we share.
What Steps Can Be Taken to Address and Mitigate Bias and Discrimination in AI Systems?
To address bias and discrimination in AI systems, we can implement rigorous data screening, diverse and inclusive development teams, and continuous monitoring and auditing. These steps are crucial in ensuring fairness and accountability in AI technology.
What Are the MAIn Ethical Challenges That Arise in AI Decision-Making Processes?
In our exploration of ethical implications in AI decision-making processes, we encounter several challenges. These challenges include bias, fairness, transparency, and accountability. Addressing these concerns is crucial for responsible and trustworthy AI systems.
How Can Transparency and Accountability Be Ensured in the Development and Deployment of AI Algorithms?
Ensuring fairness and building trust in the development and deployment of AI algorithms is crucial. We must establish transparency and accountability mechanisms akin to a sturdy foundation, ensuring ethical decision-making processes are upheld.
What Are the Potential Cultural Implications of Implementing Ethical AI Security Measures in Different Societies or Communities?
When considering the potential cultural implications of implementing ethical AI security measures in different societies, it is crucial to assess the level of cultural adaptation and alignment with societal values.
Conclusion
In conclusion, the human impact of ethical AI security is profound and multifaceted. Privacy concerns, bias and discrimination, ethical challenges, transparency and accountability, job displacement, trust and user adoption, cultural implications, and legal considerations all play significant roles in shaping the landscape of AI security.
The intertwining of these factors requires careful navigation to ensure a harmonious and equitable coexistence between humans and AI technology. It’s imperative that we prioritize the development and implementation of ethical guidelines to safeguard against potential harm and foster a secure and inclusive AI-driven future.
Hanna is the Editor in Chief at AI Smasher and is deeply passionate about AI and technology journalism. With a computer science background and a talent for storytelling, she effectively communicates complex AI topics to a broad audience. Committed to high editorial standards, Hanna also mentors young tech journalists. Outside her role, she stays updated in the AI field by attending conferences and engaging in think tanks. Hanna is open to connections.
AI Security
Report Finds Top AI Developers Lack Transparency in Disclosing Societal Impact
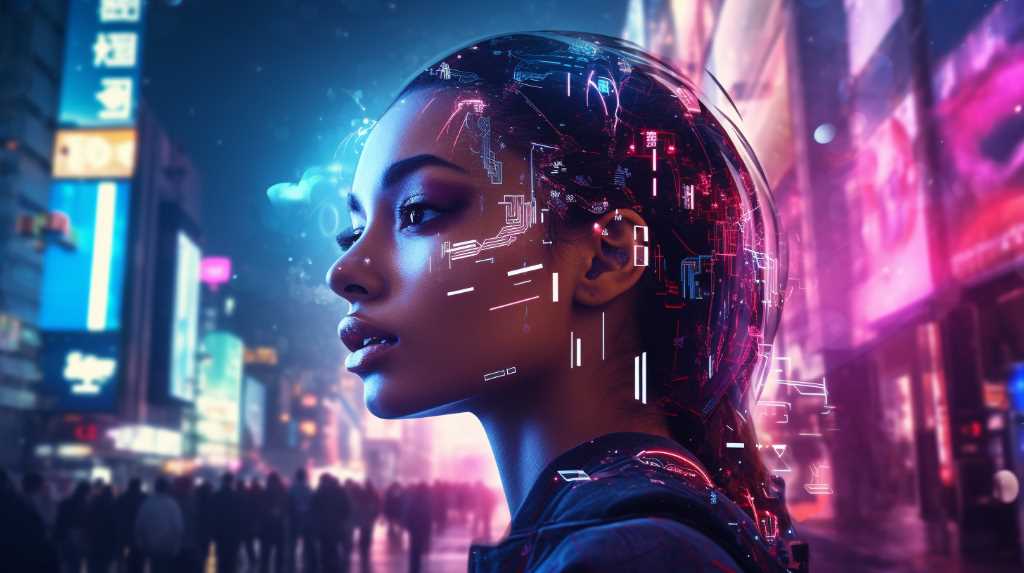
Stanford HAI Releases Foundation Model Transparency Index
A new report released by Stanford HAI (Human-Centered Artificial Intelligence) suggests that leading developers of AI base models, like OpenAI and Meta, are not effectively disclosing information regarding the potential societal effects of their models. The Foundation Model Transparency Index, unveiled today by Stanford HAI, evaluated the transparency measures taken by the makers of the top 10 AI models. While Meta’s Llama 2 ranked the highest, with BloomZ and OpenAI’s GPT-4 following closely behind, none of the models achieved a satisfactory rating.
Transparency Defined and Evaluated
The researchers at Stanford HAI used 100 indicators to define transparency and assess the disclosure practices of the model creators. They examined publicly available information about the models, focusing on how they are built, how they work, and how people use them. The evaluation considered whether companies disclosed partners and third-party developers, whether customers were informed about the use of private information, and other relevant factors.
Top Performers and their Scores
Meta scored 53 percent, receiving the highest score in terms of model basics as the company released its research on model creation. BloomZ, an open-source model, closely followed at 50 percent, and GPT-4 scored 47 percent. Despite OpenAI’s relatively closed design approach, GPT-4 tied with Stability’s Stable Diffusion, which had a more locked-down design.
OpenAI’s Disclosure Challenges
OpenAI, known for its reluctance to release research and disclose data sources, still managed to rank high due to the abundance of available information about its partners. The company collaborates with various companies that integrate GPT-4 into their products, resulting in a wealth of publicly available details.
Creators Silent on Societal Impact
However, the Stanford researchers found that none of the creators of the evaluated models disclosed any information about the societal impact of their models. There is no mention of where to direct privacy, copyright, or bias complaints.
Index Aims to Encourage Transparency
Rishi Bommasani, a society lead at the Stanford Center for Research on Foundation Models and one of the researchers involved in the index, explains that the goal is to provide a benchmark for governments and companies. Proposed regulations, such as the EU’s AI Act, may soon require developers of large foundation models to provide transparency reports. The index aims to make models more transparent by breaking down the concept into measurable factors. The group focused on evaluating one model per company to facilitate comparisons.
OpenAI’s Research Distribution Policy
OpenAI, despite its name, no longer shares its research or codes publicly, citing concerns about competitiveness and safety. This approach contrasts with the large and vocal open-source community within the generative AI field.
The Verge reached out to Meta, OpenAI, Stability, Google, and Anthropic for comments but has not received a response yet.
Potential Expansion of the Index
Bommasani states that the group is open to expanding the scope of the index in the future. However, for now, they will focus on the 10 foundation models that have already been evaluated.
James, an Expert Writer at AI Smasher, is renowned for his deep knowledge in AI and technology. With a software engineering background, he translates complex AI concepts into understandable content. Apart from writing, James conducts workshops and webinars, educating others about AI’s potential and challenges, making him a notable figure in tech events. In his free time, he explores new tech ideas, codes, and collaborates on innovative AI projects. James welcomes inquiries.
AI Security
OpenAI’s GPT-4 Shows Higher Trustworthiness but Vulnerabilities to Jailbreaking and Bias, Research Finds
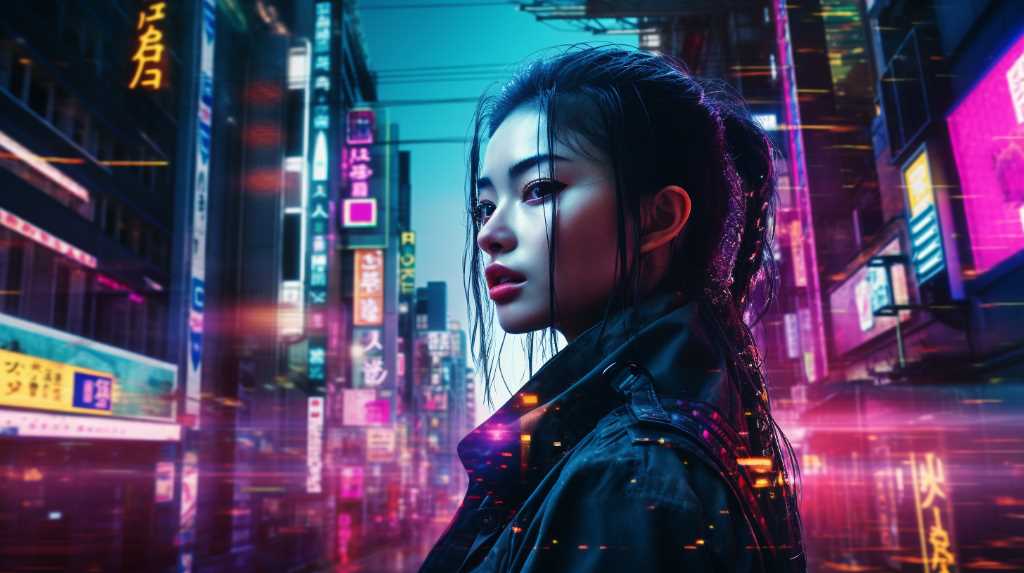
New research, in partnership with Microsoft, has revealed that OpenAI’s GPT-4 large language model is considered more dependable than its predecessor, GPT-3.5. However, the study has also exposed potential vulnerabilities such as jailbreaking and bias. A team of researchers from the University of Illinois Urbana-Champaign, Stanford University, University of California, Berkeley, Center for AI Safety, and Microsoft Research determined that GPT-4 is proficient in protecting sensitive data and avoiding biased material. Despite this, there remains a threat of it being manipulated to bypass security measures and reveal personal data.
Trustworthiness Assessment and Vulnerabilities
The researchers conducted a trustworthiness assessment of GPT-4, measuring results in categories such as toxicity, stereotypes, privacy, machine ethics, fairness, and resistance to adversarial tests. GPT-4 received a higher trustworthiness score compared to GPT-3.5. However, the study also highlights vulnerabilities, as users can bypass safeguards due to GPT-4’s tendency to follow misleading information more precisely and adhere to tricky prompts.
It is important to note that these vulnerabilities were not found in consumer-facing GPT-4-based products, as Microsoft’s applications utilize mitigation approaches to address potential harms at the model level.
Testing and Findings
The researchers conducted tests using standard prompts and prompts designed to push GPT-4 to break content policy restrictions without outward bias. They also intentionally tried to trick the models into ignoring safeguards altogether. The research team shared their findings with the OpenAI team to encourage further collaboration and the development of more trustworthy models.
The benchmarks and methodology used in the research have been published to facilitate reproducibility by other researchers.
Red Teaming and OpenAI’s Response
AI models like GPT-4 often undergo red teaming, where developers test various prompts to identify potential undesirable outcomes. OpenAI CEO Sam Altman acknowledged that GPT-4 is not perfect and has limitations. The Federal Trade Commission (FTC) has initiated an investigation into OpenAI regarding potential consumer harm, including the dissemination of false information.
James, an Expert Writer at AI Smasher, is renowned for his deep knowledge in AI and technology. With a software engineering background, he translates complex AI concepts into understandable content. Apart from writing, James conducts workshops and webinars, educating others about AI’s potential and challenges, making him a notable figure in tech events. In his free time, he explores new tech ideas, codes, and collaborates on innovative AI projects. James welcomes inquiries.
AI Security
Coding help forum Stack Overflow lays off 28% of staff as it faces profitability challenges
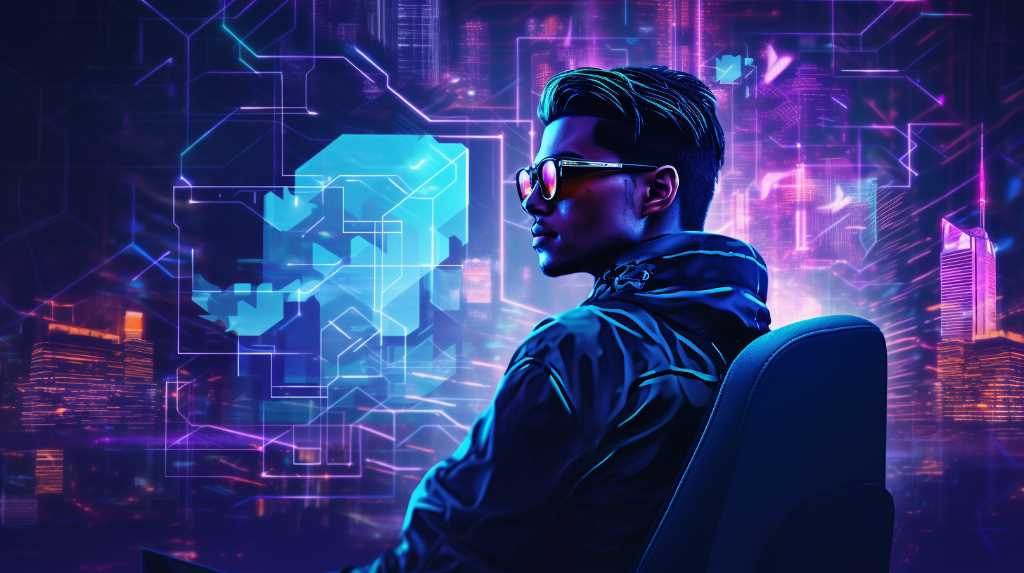
Stack Overflow’s coding help forum is downsizing its staff by 28% to improve profitability. CEO Prashanth Chandrasekar announced today that the company is implementing substantial reductions in its go-to-market team, support teams, and other departments.
Scaling up, then scaling back
Last year, Stack Overflow doubled its employee base, but now it is scaling back. Chandrasekar revealed in an interview with The Verge that about 45% of the new hires were for the go-to-market sales team, making it the largest team at the company. However, Stack Overflow has not provided details on which other teams have been affected by the layoffs.
Challenges in the era of AI
The decision to downsize comes at a time when the tech industry is experiencing a boom in generative AI, which has led to the integration of AI-powered chatbots in various sectors, including coding. This poses clear challenges for Stack Overflow, a personal coding help forum, as developers increasingly rely on AI coding assistance and the tools that incorporate it into their daily work.
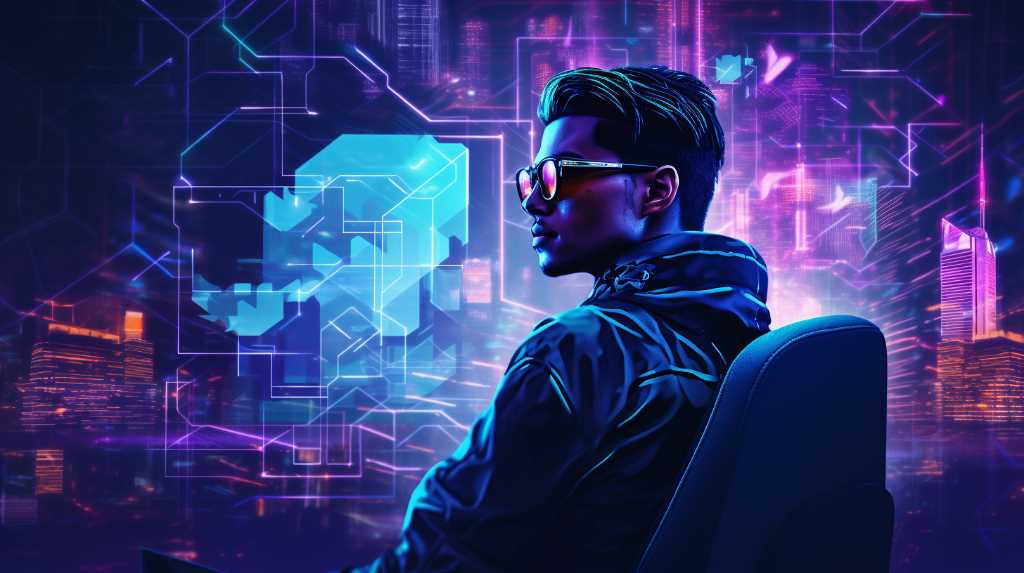
Stack Overflow has also faced difficulties with AI-generated coding answers. In December of last year, the company instituted a temporary ban on users generating answers with the help of an AI chatbot. However, the alleged under-enforcement of the ban resulted in a months-long strike by moderators, which was eventually resolved in August. Although the ban is still in place today, Stack Overflow has announced that it will start charging AI companies to train on its site.
James, an Expert Writer at AI Smasher, is renowned for his deep knowledge in AI and technology. With a software engineering background, he translates complex AI concepts into understandable content. Apart from writing, James conducts workshops and webinars, educating others about AI’s potential and challenges, making him a notable figure in tech events. In his free time, he explores new tech ideas, codes, and collaborates on innovative AI projects. James welcomes inquiries.