AI in Business
9 Essential Tips for Mastering Marketing Automation With Machine Learning
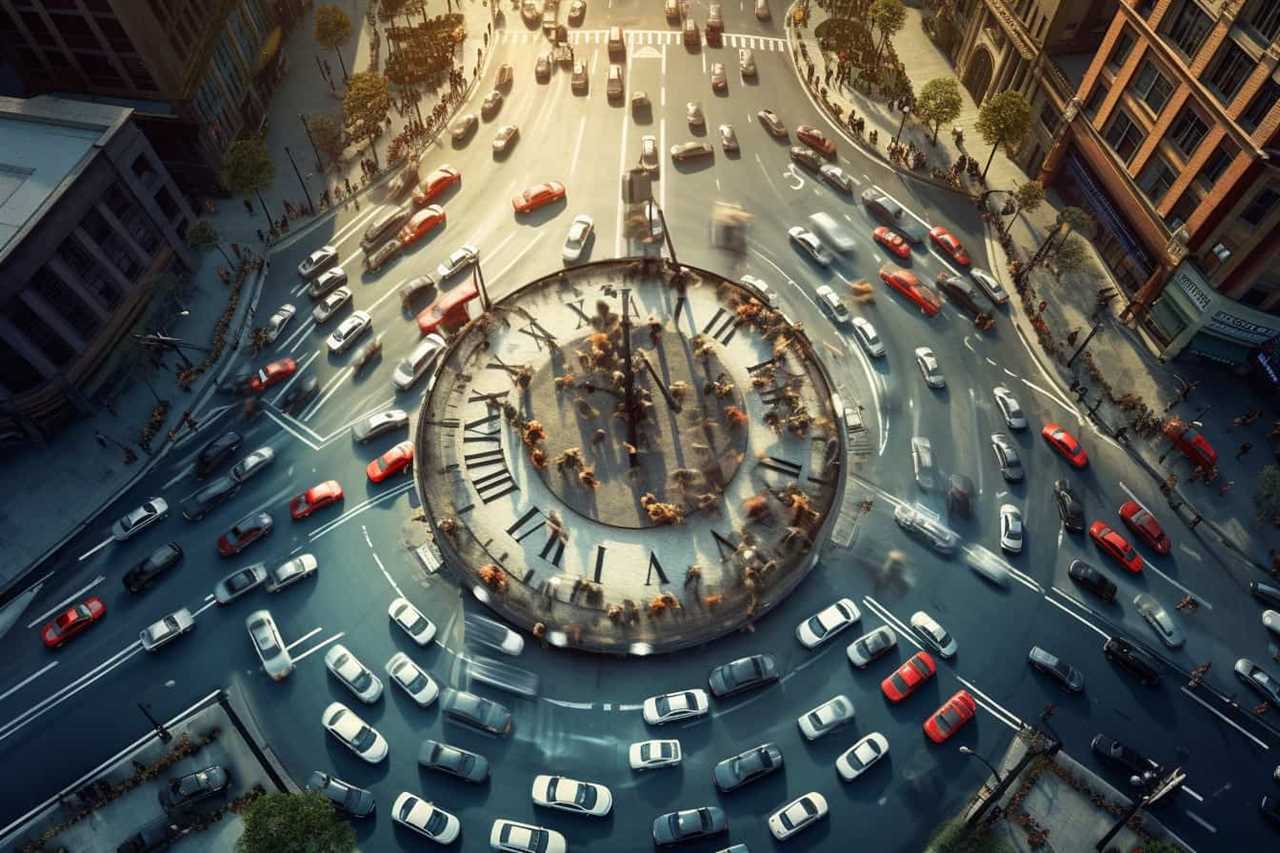
Are you ready to enhance your marketing automation strategies?
We’ve got 9 essential tips to help you master marketing automation with machine learning.
From understanding the basics to optimizing customer segmentation, leveraging predictive analytics, and automating customer journey mapping, we’ll guide you through the process of using machine learning to enhance your marketing strategies.
Get ready to see measurable results and continuous improvement with these expert tips.
Let’s dive in!
Key Takeaways
- Machine learning has various applications in marketing automation, such as predictive analytics and recommendation systems.
- Choosing the right marketing automation platform is crucial, considering features, integration capabilities, scalability, and alignment with marketing goals.
- Proper data collection, cleaning, and feature engineering are fundamental for successful machine learning implementation in marketing automation.
- Implementing machine learning algorithms for personalization can drive customer engagement and boost conversion rates.
Understanding the Basics of Machine Learning
We begin by delving into the fundamentals of machine learning, focusing on the key aspects and principles that underpin its functionality. Understanding machine learning applications is essential for mastery in this field.
Machine learning is a branch of artificial intelligence that enables computers to learn from data without being explicitly programmed. It has a wide range of applications, from image recognition and natural language processing to predictive analytics and recommendation systems.
Evaluating machine learning models is crucial in ensuring their effectiveness and accuracy. This involves assessing their performance metrics, such as accuracy, precision, recall, and F1 score. Additionally, it’s important to consider factors like the quality and quantity of training data, feature engineering, and model complexity.
Identifying the Right Marketing Automation Platform
Continuing our exploration of machine learning in marketing automation, let’s now focus on identifying the ideal marketing automation platform.
When it comes to choosing the right marketing automation tools, it’s crucial to evaluate various marketing automation platforms to find the best fit for your business. Here are three key factors to consider:
-
Features and functionality: Look for a platform that offers a wide range of features, such as lead scoring, email marketing automation, campaign management, and customer segmentation. Ensure that the platform aligns with your marketing goals and supports your specific requirements.
-
Integration capabilities: Evaluate the platform’s integration capabilities with your existing tech stack. It should seamlessly integrate with your CRM, customer support system, and other tools to create a unified ecosystem that enhances your marketing efforts.
-
Scalability and flexibility: Choose a platform that can grow with your business and adapt to your changing needs. It should allow you to easily scale your marketing automation efforts and accommodate future expansion plans.
Collecting and Preparing Data for Machine Learning
To effectively utilize machine learning in marketing automation, data collection and preparation are essential steps. Before applying machine learning algorithms, it’s crucial to ensure the data is accurate, consistent, and relevant.
This involves a process called data cleaning, where inconsistencies, errors, and missing values are identified and corrected. Additionally, feature engineering plays a vital role in preparing the data for machine learning. It involves selecting and creating relevant features that will help the algorithms make accurate predictions.
By transforming and optimizing the features, the models can better understand patterns and relationships within the data. Proper data cleaning and feature engineering are fundamental for successful machine learning implementation in marketing automation.
Now, let’s explore the next step: implementing machine learning algorithms for personalization.
Implementing Machine Learning Algorithms for Personalization
When it comes to implementing machine learning algorithms for personalization, there are several key points to consider.
First, the benefits of personalization can’t be overstated – it allows for a more tailored and engaging customer experience, which can lead to increased conversions and customer loyalty.
Second, effective algorithm selection is crucial, as different algorithms have varying strengths and weaknesses in terms of their ability to handle different types of data and tasks.
Benefits of Personalization
How can we effectively implement machine learning algorithms for personalization to reap the benefits?
Implementing machine learning algorithms for personalization can bring numerous benefits to businesses. Here are three key advantages of personalization:
-
Increased customer engagement: By tailoring marketing messages and experiences to each individual customer, businesses can capture their attention and create a deeper level of engagement. Personalized content resonates with customers, making them more likely to interact with your brand and stay connected.
-
Improved conversion rates: Personalization enables businesses to deliver relevant offers and recommendations to customers, increasing the chances of conversions. When customers feel understood and valued, they’re more likely to make a purchase or take the desired action.
-
Enhanced customer satisfaction: Personalization shows customers that you understand their needs and preferences, leading to increased customer satisfaction. By providing personalized experiences, businesses can foster long-term relationships and loyalty.
Implementing machine learning algorithms for personalization can be a game-changer for businesses, driving customer engagement, improving conversion rates, and boosting overall customer satisfaction.
Effective Algorithm Selection
In order to effectively implement machine learning algorithms for personalization, we must carefully select the most suitable algorithm for our specific marketing automation needs. This requires evaluating the algorithm performance and conducting model evaluation to ensure optimal results. When choosing an algorithm, it is essential to consider factors such as the size and quality of the dataset, the complexity of the problem, and the computational resources available. To facilitate the decision-making process, a table comparing different algorithms can be useful:
Algorithm | Pros | Cons |
---|---|---|
Decision Trees | Easy to interpret, handle non-linear relationships | Prone to overfitting, sensitive to small changes |
Random Forest | Good for feature selection, robust against overfitting | Computationally expensive, lack of interpretability |
Support Vector Machines | Effective for high-dimensional datasets, good generalization ability | Can be slow for large datasets, sensitive to parameter tuning |
Neural Networks | Powerful for complex problems, can learn non-linear relationships | Require large amounts of data, difficult to interpret |
Data Privacy Considerations
Our first consideration when implementing machine learning algorithms for personalization is ensuring the privacy of our data. In order to achieve this, we need to abide by data privacy regulations and address ethical considerations. Here are three key points to consider:
-
Compliance with data privacy regulations: We must ensure that our data collection, storage, and usage practices align with relevant regulations such as the General Data Protection Regulation (GDPR) and the California Consumer Privacy Act (CCPA). This includes obtaining proper consent, implementing security measures, and providing individuals with control over their personal data.
-
Transparency and accountability: It’s essential to be transparent with users about the data we collect and how it’s used. Clear privacy policies and consent mechanisms should be in place, and individuals should have the ability to opt out or modify their preferences. Additionally, we should establish accountability by regularly auditing our data practices and ensuring compliance with ethical guidelines.
-
Minimizing data collection and retention: To prioritize data privacy, we should only collect and retain the minimum amount of personal data necessary for personalization. By implementing data minimization practices, we can reduce the risk of unauthorized access or misuse of personal information.
Optimizing Customer Segmentation With Machine Learning
When it comes to optimizing customer segmentation with machine learning, there are two key benefits: improved targeting accuracy and personalized customer experiences.
Improved Targeting Accuracy
To optimize customer segmentation with machine learning, we rely on the power of machine learning algorithms to enhance targeting accuracy. By leveraging machine learning, we can significantly improve our ability to identify and reach the right customers at the right time.
Here are three ways machine learning can help us achieve improved targeting accuracy:
-
Predictive modeling: Machine learning algorithms can analyze vast amounts of customer data to identify patterns and trends. This enables us to create predictive models that accurately forecast customer behavior, increasing conversion rates.
-
Personalization: Machine learning algorithms can analyze individual customer data to create personalized marketing messages and offers. This level of personalization not only improves targeting accuracy but also enhances customer engagement and satisfaction, maximizing ROI.
-
Real-time optimization: With machine learning, we can continuously analyze customer data in real-time, allowing us to adapt and optimize our marketing campaigns on the fly. This dynamic approach ensures that our targeting is always up-to-date and relevant, further increasing conversion and maximizing ROI.
Personalized Customer Experiences
Using machine learning, we can optimize customer segmentation and create personalized customer experiences that enhance targeting accuracy and drive engagement. By analyzing large volumes of customer data, machine learning algorithms can identify patterns and preferences, allowing marketers to tailor their messaging and offerings to individual customers.
This level of personalization not only improves customer satisfaction but also increases the effectiveness of targeted advertising campaigns. With machine learning, marketers can identify the most relevant customer segments for a specific campaign and deliver personalized content that resonates with each segment.
This not only improves the chances of conversion but also enhances customer loyalty and retention. By leveraging machine learning for personalized customer experiences, businesses can create more meaningful connections with their customers and drive long-term success.
Now, let’s explore how leveraging predictive analytics can further enhance targeted marketing efforts.
Leveraging Predictive Analytics for Targeted Marketing
We leverage predictive analytics to enhance targeted marketing. By using predictive modeling, we can analyze large amounts of data to identify patterns and trends in customer behavior. This allows us to make accurate predictions about future customer actions and preferences. With customer behavior analysis, we gain insights into individual customer journeys, enabling us to create personalized marketing campaigns that resonate with each customer.
Here are three ways we leverage predictive analytics for targeted marketing:
-
Segmentation: We divide our customer base into different segments based on their characteristics and behaviors. This helps us tailor our marketing messages and offers to specific groups, increasing the likelihood of engagement and conversion.
-
Recommendation engines: By analyzing customer data and behavior, we can create recommendation engines that suggest products or content based on individual preferences. This enhances the customer experience and increases the chances of cross-selling or upselling.
-
Churn prediction: Through predictive analytics, we can identify customers who are at risk of churning. This allows us to take proactive measures to retain them, such as targeted offers or personalized communication.
Enhancing Lead Scoring With Machine Learning
When it comes to mastering marketing automation with machine learning, one essential aspect to consider is enhancing lead scoring through the utilization of advanced algorithms.
By leveraging machine learning techniques, marketers can improve their conversion rates by accurately identifying and prioritizing leads based on their likelihood to convert. Machine learning algorithms can analyze vast amounts of data, such as customer behavior and interactions, to identify patterns and trends that indicate the likelihood of a lead becoming a customer.
These algorithms can also take into account various factors, such as demographics, purchase history, and engagement levels, to assign a lead score that reflects their potential value.
Automating Customer Journey Mapping With Machine Learning
To automate customer journey mapping with machine learning, our team can leverage advanced algorithms to analyze customer interactions and behavior, allowing us to gain valuable insights and optimize the customer experience.
Here are three ways in which automating customer journey mapping can benefit our marketing efforts:
-
Improved personalization: By analyzing customer interactions and behavior, machine learning algorithms can identify patterns and preferences, enabling us to deliver personalized marketing messages and offers at the right time and through the right channels.
-
Automating sales forecasting: Machine learning algorithms can analyze customer journey data to predict future sales and identify potential upsell and cross-sell opportunities. This helps us make data-driven decisions and allocate resources more effectively.
-
Enhancing customer retention: By understanding the customer journey and identifying pain points or areas of improvement, machine learning can help us develop targeted retention strategies. This includes personalized recommendations, loyalty programs, and proactive customer service, ultimately increasing customer satisfaction and loyalty.
Measuring and Analyzing Results for Continuous Improvement
For ongoing improvement, we measure and analyze the results of our marketing automation efforts using machine learning. By continuously evaluating our performance, we can identify areas for improvement and make data-driven decisions to optimize our marketing strategies. To effectively measure and analyze our results, we utilize various metrics and techniques.
Here is a table showcasing some of the key metrics we track and evaluate:
Metric | Description |
---|---|
Conversion Rate | Measures the percentage of visitors who take the desired action, such as making a purchase or subscribing to a newsletter. |
Customer Lifetime Value | Calculates the projected revenue a customer will generate over their entire relationship with the company. |
Email Open Rate | Measures the percentage of recipients who open our marketing emails, indicating the effectiveness of our subject lines and email content. |
Return on Investment | Evaluates the profitability of our marketing campaigns by comparing the revenue generated to the cost of the campaign. |
Frequently Asked Questions
How Can Machine Learning Enhance Lead Scoring in Marketing Automation?
Machine learning enhances lead scoring in marketing automation by applying advanced algorithms to analyze customer data. It improves customer segmentation, allowing us to target the right audience with personalized content, resulting in higher conversion rates and ROI.
What Are Some Common Challenges in Automating Customer Journey Mapping With Machine Learning?
Challenges in automating customer journey mapping with machine learning include data integration, model accuracy, and interpretation of results. Solutions involve ensuring data quality, refining models, and developing a deep understanding of the customer journey.
How Can Predictive Analytics Be Leveraged for Targeted Marketing?
Predictive analytics allows us to develop data-driven strategies for targeted marketing. By leveraging machine learning, we can identify patterns and make accurate predictions, resulting in increased efficiency, better customer engagement, and improved ROI.
What Are the Best Practices for Measuring and Analyzing Results in Marketing Automation With Machine Learning?
Measuring effectiveness and analyzing data are crucial for mastering marketing automation with machine learning. We must understand the impact of our strategies and make data-driven decisions to ensure success in this complex field.
How Can Machine Learning Algorithms Be Implemented for Personalization in Marketing Automation?
Machine learning algorithms can greatly enhance personalization in marketing automation. By using these algorithms in email campaigns, we can deliver targeted content based on customer segmentation, resulting in higher engagement and conversion rates.
Conclusion
In the vast ocean of marketing, machine learning serves as our compass, guiding us towards success. By harnessing its power, we can navigate through the complexities of customer segmentation, predictive analytics, and personalized marketing.
Just as a skilled captain relies on the stars to chart their course, we must embrace machine learning as our guiding light. With its ability to automate processes and analyze data, we can sail towards continuous improvement and reach new horizons of marketing success.
Hanna is the Editor in Chief at AI Smasher and is deeply passionate about AI and technology journalism. With a computer science background and a talent for storytelling, she effectively communicates complex AI topics to a broad audience. Committed to high editorial standards, Hanna also mentors young tech journalists. Outside her role, she stays updated in the AI field by attending conferences and engaging in think tanks. Hanna is open to connections.
AI in Business
Unveiling the Power of Sentiment Analysis in AI for Business
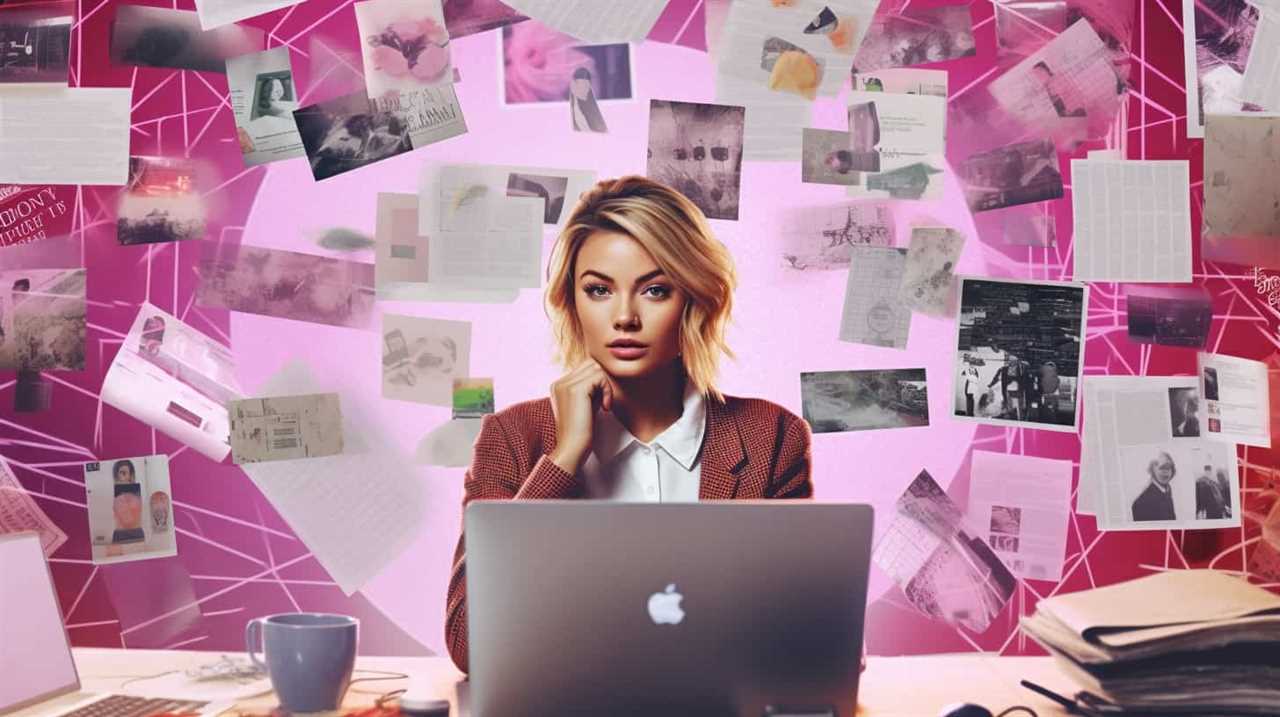
We made an exciting discovery: Sentiment analysis is revolutionizing AI for businesses.
Did you know that 85% of customers trust online reviews as much as personal recommendations?
In this article, we’ll delve into the power of sentiment analysis in the world of business AI. By harnessing the capabilities of natural language processing, sentiment analysis enables companies to analyze and interpret customer opinions and emotions.
Join us as we explore the benefits, techniques, applications, challenges, and future directions of sentiment analysis for business AI.
Key Takeaways
- Sentiment analysis helps businesses understand customer attitudes and opinions towards their products or services.
- Natural Language Processing (NLP) algorithms and machine learning models are used in sentiment analysis to analyze and interpret large amounts of text data.
- NLP enables businesses to efficiently process and understand social media sentiment, providing real-time monitoring and reliable insights.
- Sentiment analysis has real-world applications in monitoring public sentiment, improving customer satisfaction, and aiding in reputation management.
Overview of Sentiment Analysis in Business AI
In our article, we’ll provide a comprehensive overview of the role and significance of sentiment analysis in AI for businesses.
Sentiment analysis is a powerful technique that helps organizations extract valuable insights from customer feedback and online conversations. By analyzing sentiment, companies can understand customer opinions, emotions, and attitudes towards their products or services.
There are various sentiment analysis techniques available, including rule-based, machine learning, and hybrid approaches. These techniques involve the use of sentiment analysis tools, such as Natural Language Processing (NLP) algorithms, lexicons, and machine learning models. These tools help automate the process of sentiment analysis and can handle large volumes of data efficiently.
With sentiment analysis, businesses can make data-driven decisions, improve customer satisfaction, identify emerging trends, and enhance their overall brand reputation.
Benefits of Natural Language Processing in Sentiment Analysis
One significant benefit of Natural Language Processing (NLP) in sentiment analysis is its ability to accurately analyze and interpret large amounts of text data. NLP techniques enable businesses to efficiently process and understand social media sentiment, allowing them to gain valuable insights into public opinion and customer satisfaction.
Some benefits of NLP in sentiment analysis include:
-
Improved accuracy: NLP algorithms can identify and analyze sentiment with a high degree of precision and accuracy, providing businesses with reliable insights.
-
Efficient data processing: NLP enables businesses to process and analyze large volumes of text data in a relatively short amount of time, saving valuable resources.
-
Real-time monitoring: NLP techniques allow businesses to track social media sentiment in real-time, enabling them to respond promptly to customer feedback and concerns.
-
Enhanced decision-making: By leveraging NLP in sentiment analysis, businesses can make data-driven decisions based on a comprehensive understanding of customer sentiment and preferences.
Techniques for Training Sentiment Analysis Models
To effectively train sentiment analysis models, we employ various techniques that allow us to extract meaningful insights from large amounts of textual data.
One popular technique is transfer learning, which involves leveraging pre-trained models on vast datasets and fine-tuning them for specific sentiment analysis tasks. By using transfer learning, we can benefit from the knowledge and patterns learned by these models on general language understanding, saving time and resources.
Another technique is feature extraction, where we extract relevant features from the text that can help us classify sentiment. These features can include word frequencies, n-grams, or even more complex linguistic features like sentiment lexicons. By selecting and extracting the right features, we can enhance the accuracy and performance of our sentiment analysis models, making them more effective in understanding and classifying sentiment in textual data.
Real-world Applications of Sentiment Analysis in Business AI
Using transfer learning and feature extraction techniques, we can now explore real-world applications of sentiment analysis in business AI.
Sentiment analysis in social media allows companies to monitor and analyze public sentiment towards their brand, products, and services. By analyzing social media posts, comments, and reviews, businesses can gain valuable insights into customer opinions and identify trends and patterns.
Sentiment analysis in customer reviews helps companies understand customer satisfaction levels and identify areas for improvement. It enables companies to proactively address any negative feedback and improve customer experience.
Additionally, sentiment analysis can be used for reputation management, allowing businesses to track and manage their online reputation by identifying and addressing any negative sentiment.
Challenges and Future Directions in Sentiment Analysis for Business AI
As we delve into the challenges and future directions of sentiment analysis for business AI, we continue to explore its applications and the potential it holds for enhancing customer satisfaction and strategic decision-making.
Looking ahead, future advancements in sentiment analysis will likely focus on improving accuracy and reliability. This includes developing more sophisticated algorithms that can better understand complex emotions and subtle nuances in text. Additionally, advancements in natural language processing and machine learning techniques will enable sentiment analysis systems to become more context-aware, taking into account factors such as sarcasm and irony.
However, with these advancements also come ethical implications. As sentiment analysis becomes more powerful and pervasive, there’s a need to address issues of privacy, bias, and the responsible use of customer data. Striking the right balance between innovation and ethical considerations will be crucial for the future of sentiment analysis in business AI.
Frequently Asked Questions
Can Sentiment Analysis Be Used in Industries Other Than Business Ai?
Sentiment analysis can indeed be applied in industries beyond business AI. For instance, sentiment analysis finds use in healthcare by analyzing patient feedback. Furthermore, it is employed in political campaigns to gauge public opinion and tailor messaging accordingly.
What Are the Limitations of Using Natural Language Processing in Sentiment Analysis?
Sentiment analysis accuracy and challenges in natural language processing include bias, sarcasm, and context understanding. These limitations hinder the ability to accurately interpret emotions, making sentiment analysis a complex task in AI for business.
How Long Does It Typically Take to Train a Sentiment Analysis Model?
Training time for sentiment analysis models varies depending on the dataset size and complexity. Generally, it takes a few hours to a few days. However, longer training times can lead to higher model accuracy, ensuring reliable sentiment analysis results.
Are There Any Ethical Concerns Surrounding the Use of Sentiment Analysis in Business Ai?
There are ethical implications and privacy concerns surrounding the use of sentiment analysis in business AI. We must consider the potential misuse of personal data and the potential for biased or discriminatory outcomes.
What Are the Potential Future Advancements and Developments in Sentiment Analysis for Business Ai?
In the future, we can expect exciting advancements and developments in sentiment analysis for business AI. AI technologies will continue to evolve, enabling more accurate and nuanced analysis of sentiment, leading to better decision-making and customer insights.
Conclusion
In conclusion, sentiment analysis holds immense potential in shaping the future of AI for business. By harnessing the power of natural language processing techniques, organizations can gain valuable insights into customer sentiments, enabling them to make data-driven decisions.
However, like any evolving technology, sentiment analysis faces challenges and requires continual advancements. As the field progresses, it’s crucial for researchers and practitioners to address these challenges and explore new avenues to further enhance the accuracy and applicability of sentiment analysis in the realm of business AI.
Hanna is the Editor in Chief at AI Smasher and is deeply passionate about AI and technology journalism. With a computer science background and a talent for storytelling, she effectively communicates complex AI topics to a broad audience. Committed to high editorial standards, Hanna also mentors young tech journalists. Outside her role, she stays updated in the AI field by attending conferences and engaging in think tanks. Hanna is open to connections.
AI in Business
Why Natural Language Processing Is a Game-Changer for Customer Experience
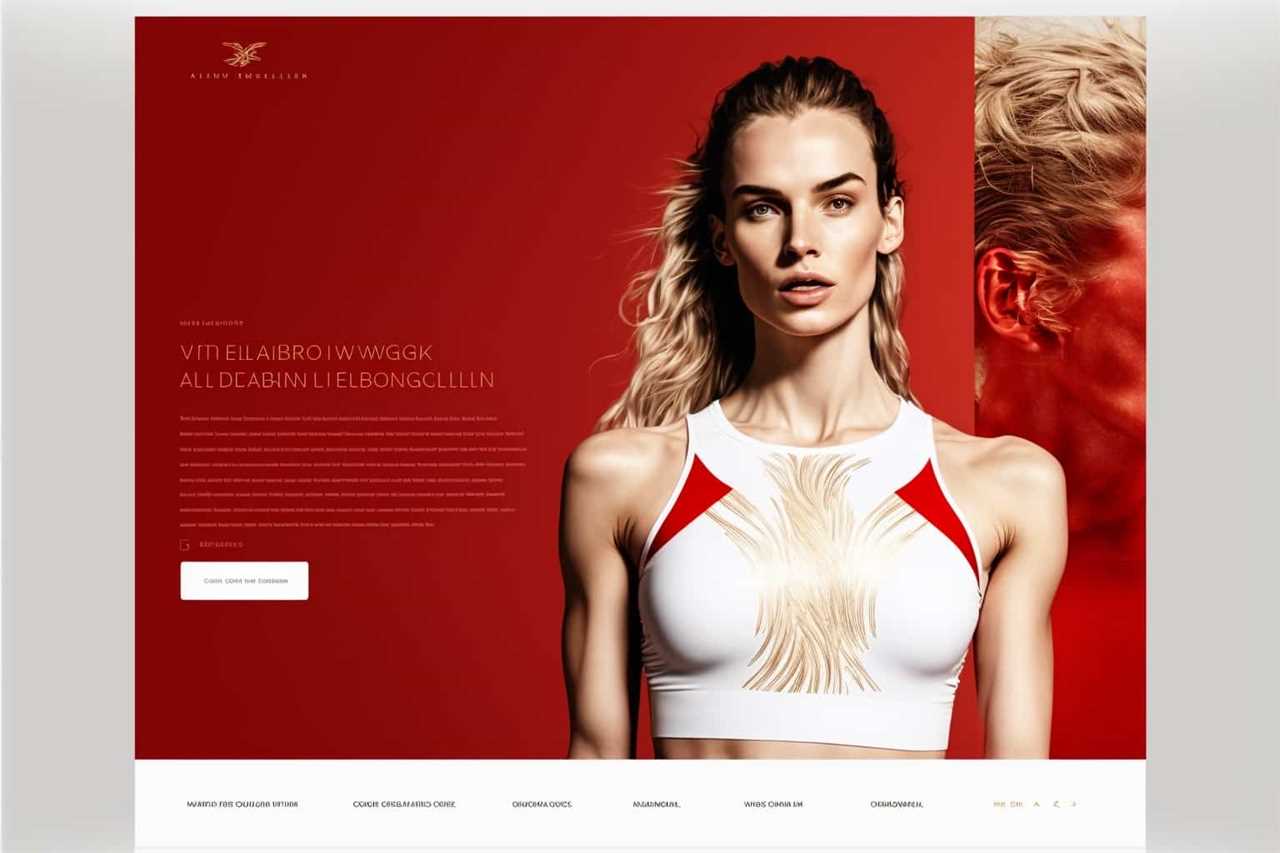
Imagine a situation where customer interactions are improved, understanding of customer needs is increased, and customer support becomes more effective. Enter the world of Natural Language Processing (NLP).
With NLP technology, we can transform customer experiences into personalized journeys. Through the power of language processing, we can unlock a new level of customer satisfaction.
In this article, we will explore why NLP is revolutionizing the way businesses connect with their customers. Get ready to embark on a journey towards mastery in customer experience.
Key Takeaways
- AI chatbots revolutionize customer service by providing automated support.
- Natural language processing enables accurate interpretation of customer intents.
- NLP technology helps businesses enhance their services according to customer needs.
- NLP technology contributes to a positive customer experience.
Enhanced Customer Interactions Through AI
We have witnessed a significant improvement in customer interactions through the implementation of AI technologies.
AI powered chatbots have revolutionized the way businesses handle customer queries and concerns. These chatbots provide automated customer service, allowing businesses to respond to customer inquiries promptly and efficiently.
By leveraging advanced natural language processing techniques, AI chatbots can understand and interpret customer intents accurately, providing personalized and relevant responses. Additionally, sentiment analysis is used for feedback analysis, enabling businesses to gauge customer satisfaction levels and identify areas for improvement.
This valuable insight helps companies tailor their products and services to meet customer needs effectively. Furthermore, AI technologies enable proactive customer engagement, allowing businesses to anticipate customer needs and provide proactive support.
Improved Understanding of Customer Needs
By implementing natural language processing, businesses can gain a deeper understanding of their customers’ needs. This enhanced customer insights allows companies to optimize their product recommendations and deliver a more personalized experience. Natural language processing enables businesses to analyze large volumes of customer data, such as reviews, feedback, and social media interactions, to identify patterns and trends. This deeper understanding of customer needs enables businesses to tailor their products and services to meet specific demands. Through the use of advanced algorithms, businesses can generate optimized product recommendations, increasing the likelihood of customer satisfaction and loyalty. The following table illustrates how natural language processing can enhance customer insights and optimize product recommendations:
Enhanced Customer Insights | Optimized Product Recommendations | Improved Customer Experience |
---|---|---|
Analyzing customer data | Generating personalized suggestions | Meeting specific demands |
Identifying patterns and trends | Increasing customer satisfaction | Enhancing customer loyalty |
Tailoring products and services | Improving customer retention | Delivering personalized experiences |
Streamlined Customer Support With NLP
Implementing natural language processing (NLP) in customer support offers several benefits. One key advantage is the use of an automated ticketing system, which categorizes and routes customer inquiries to the appropriate department or agent. This reduces manual effort and response times, improving efficiency and ensuring faster and more accurate support for customers.
Another advantage of NLP is the integration of chatbots. These virtual assistants can understand and respond to customer queries in a conversational manner. Equipped with NLP capabilities, chatbots provide instant assistance, answer frequently asked questions, and handle basic tasks. This frees up human agents to focus on more complex customer issues.
Personalized Customer Experiences With Language Processing
One of the key benefits of language processing is its ability to provide personalized customer experiences. By analyzing customer data and understanding their preferences, businesses can tailor their marketing strategies to individual customers.
Language processing allows companies to gather valuable insights from social media platforms, enabling them to understand customer sentiment and engage with their audience effectively. With the help of language processing in social media, businesses can identify customer needs, interests, and buying behaviors, allowing them to deliver targeted and personalized marketing messages.
This not only enhances the customer experience but also increases the likelihood of conversion and customer loyalty. By utilizing language processing technologies, businesses can create personalized marketing strategies that resonate with their customers, ultimately leading to increased customer satisfaction with NLP technology.
Increased Customer Satisfaction With NLP Technology
With the implementation of natural language processing technology, we can significantly enhance customer satisfaction.
NLP applications in sales and marketing have revolutionized the way businesses interact with their customers. By analyzing and understanding customer feedback, NLP technology helps companies identify pain points and areas for improvement. This enables them to offer personalized solutions and address customer concerns more effectively.
In addition, NLP’s impact on customer loyalty can’t be underestimated. By providing real-time support and personalized recommendations, NLP technology builds trust and strengthens the customer-business relationship. Customers feel valued and understood, leading to increased satisfaction and loyalty.
Furthermore, NLP technology allows businesses to gather valuable insights from customer conversations, which can be used to improve products, services, and overall customer experience.
Frequently Asked Questions
How Does Natural Language Processing Enhance Customer Interactions Through Ai?
Natural language processing improves chatbots and enhances sentiment analysis, allowing us to better understand and respond to customer interactions. By leveraging AI, it revolutionizes customer experience by providing personalized and efficient communication.
How Does NLP Technology Improve Understanding of Customer Needs?
Improving customer interactions and enhancing customer feedback analysis, NLP technology enables us to better understand customer needs. It helps us analyze and interpret their language, leading to more personalized and effective customer experiences.
What Are the Benefits of Streamlined Customer Support With Nlp?
Efficiency and automation are the benefits of streamlined customer support with NLP. It helps us provide faster and more accurate responses, improving customer satisfaction and allowing us to handle a higher volume of inquiries.
How Does Language Processing Enable Personalized Customer Experiences?
Language processing enables personalized customer experiences by adopting a customer centric approach and providing personalized recommendations. By understanding and analyzing customer data, NLP allows us to tailor interactions and offer relevant solutions, enhancing overall satisfaction.
What Are the Ways in Which NLP Technology Increases Customer Satisfaction?
Improved communication and enhanced problem solving are some ways in which NLP technology increases customer satisfaction. It allows us to understand and respond to customer needs more effectively, leading to a more personalized and efficient customer experience.
Conclusion
In conclusion, natural language processing is a total game-changer for customer experience. With AI-enhanced interactions, businesses can better understand customer needs and provide streamlined support.
This technology allows for personalized experiences and ultimately leads to increased customer satisfaction.
So, if you want your customers to be as happy as a kid in a candy store, incorporating NLP into your customer service strategy is a no-brainer. Trust me, they’ll be singing your praises like a choir of angels.
Hanna is the Editor in Chief at AI Smasher and is deeply passionate about AI and technology journalism. With a computer science background and a talent for storytelling, she effectively communicates complex AI topics to a broad audience. Committed to high editorial standards, Hanna also mentors young tech journalists. Outside her role, she stays updated in the AI field by attending conferences and engaging in think tanks. Hanna is open to connections.
AI in Business
Humane’s AI Pin: A Closer Look at the Revolutionary Wearable
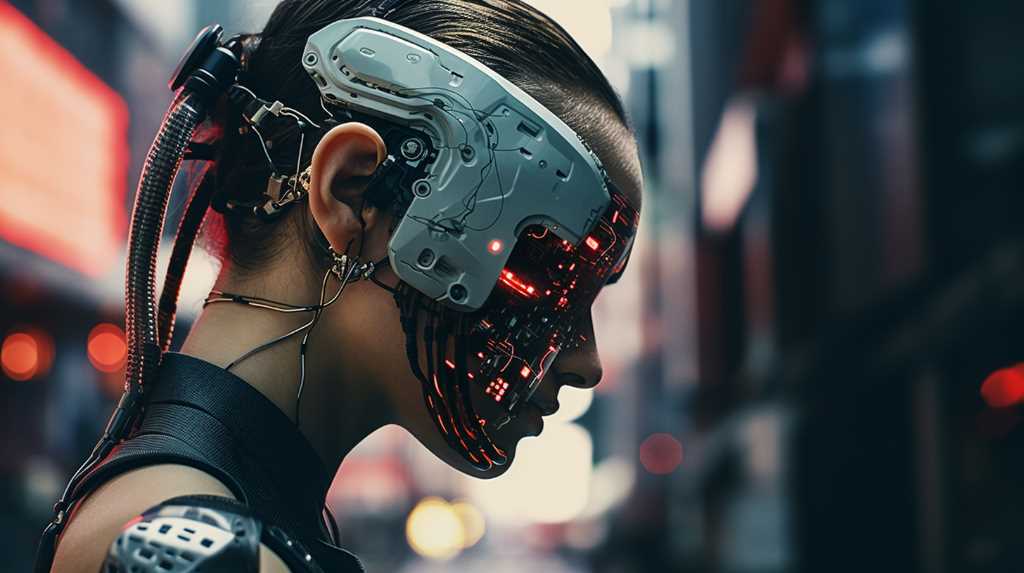
Time Magazine Recognizes AI Pin as a Top Invention of 2023
Humane, the innovative technology company, is gearing up for the launch of its highly anticipated AI Pin on November 9th. To much surprise, the device has already been honored as one of Time Magazine’s “Best Inventions of 2023,” alongside other cutting-edge gadgets such as the Framework Laptop 16 and the Samsung Galaxy Z Flip 5. This recognition has heightened the excitement surrounding the AI Pin, providing us with a more detailed look at this revolutionary wearable.
The AI Pin: A Fusion of Style and Intelligent Technology
While details have been scarce, the latest write-up provides some intriguing insights into the AI Pin. The device is designed to attach magnetically to your clothing, offering a seamless integration into your everyday life. Powered by a combination of proprietary software and OpenAI’s GPT-4, the AI Pin boasts a range of impressive features.
Trust Light: Ensuring Privacy and Transparency
One standout feature of the AI Pin is its “Trust Light.” This illuminated indicator activates whenever the device’s camera, microphone, or other sensors are recording data. This transparency aims to address concerns about privacy and data collection, setting the AI Pin apart from its competitors.
A New Kind of Wearable: The Promise of the AI Pin
Humane has been building anticipation for the AI Pin for months, starting with a captivating TED talk by co-founder Imran Chaudhri in April. Chaudhri described the AI Pin as a “new kind of wearable device and platform,” emphasizing that it operates independently of smartphones or other devices. This bold claim has left many intrigued about the inner workings and functionality of the AI Pin.
A Race to Revolutionize AI-Powered Gadgets
While Humane has generated significant buzz around its upcoming release, it faces fierce competition in the AI gadget market. Other companies, such as Rewind and a collaboration between Sam Altman and Jony Ive, are also vying to create innovative devices that harness the power of artificial intelligence. Additionally, the rapid growth of smart glasses with AI assistants, spearheaded by Meta and Amazon, has contributed to an industry-wide push to explore the potential of AI-powered hardware.
Unveiling the Future: Launching on November 9th
Despite the lingering questions surrounding its functionality, the AI Pin remains on track for its highly anticipated launch on November 9th. As the unveiling date approaches, we eagerly await a deeper understanding of what the AI Pin can do and how it will enhance our daily lives. Will this be the wearable of the future? Only time will tell.
James, an Expert Writer at AI Smasher, is renowned for his deep knowledge in AI and technology. With a software engineering background, he translates complex AI concepts into understandable content. Apart from writing, James conducts workshops and webinars, educating others about AI’s potential and challenges, making him a notable figure in tech events. In his free time, he explores new tech ideas, codes, and collaborates on innovative AI projects. James welcomes inquiries.