AI in Business
Leverage NLP in AI for Advanced Sentiment Analysis
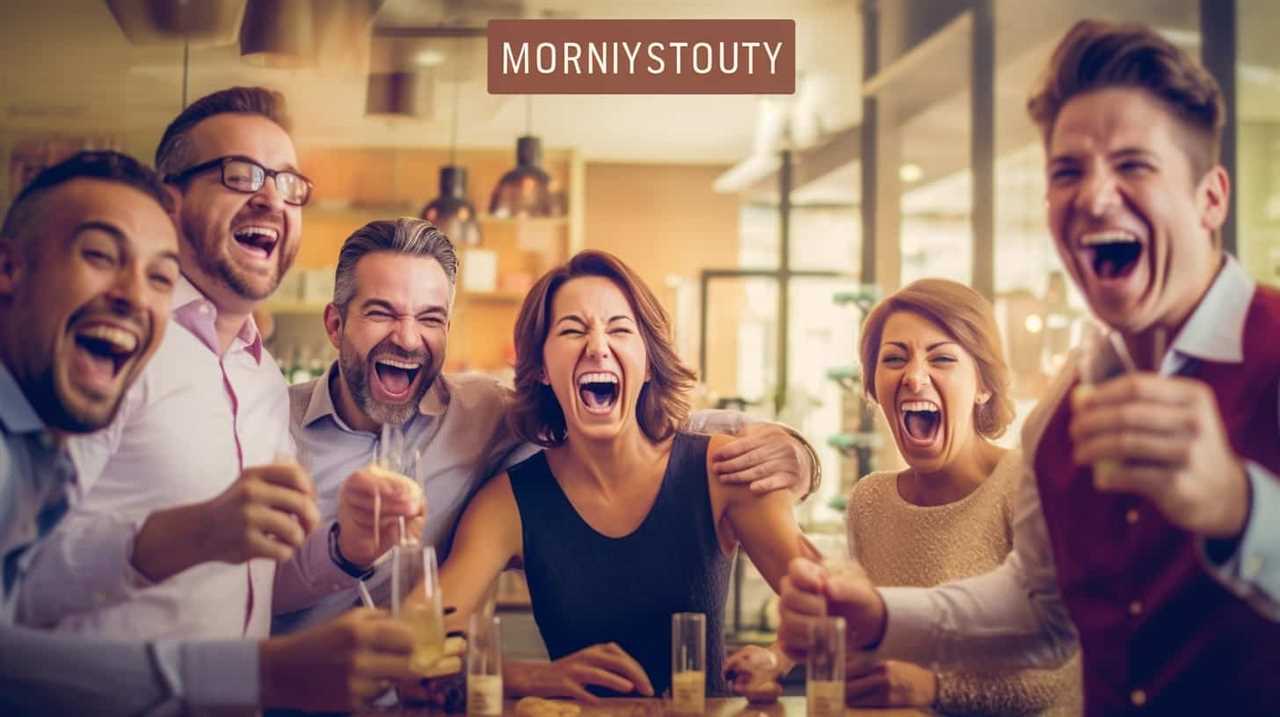
In our pursuit of mastering sentiment analysis, we harness the capabilities of Artificial Intelligence (AI) through Natural Language Processing (NLP). Through advanced analysis of text data, we reveal the genuine sentiments that are concealed within.
This article explores the key components of NLP for sentiment analysis, the challenges faced in implementation, and future trends in this field.
Join us as we dive deep into the world of NLP and unlock the full potential of sentiment analysis.
Key Takeaways
- NLP enables accurate analysis and understanding of emotions and opinions in text.
- Sentiment analysis helps companies gain insights into customer opinions and make data-driven decisions.
- Lexicon-based and machine learning approaches are commonly used for sentiment analysis.
- Advanced NLP techniques and real-time sentiment analysis are future trends in the field.
Importance of NLP in Sentiment Analysis
We believe that NLP plays a crucial role in sentiment analysis as it enables us to accurately analyze and understand the emotions and opinions expressed in text.
Sentiment analysis, a branch of natural language processing, involves the use of machine learning techniques to identify and extract subjective information from text data.
By applying NLP algorithms, we can automatically detect sentiment polarity, determine the intensity of emotions, and classify text into positive, negative, or neutral categories. This analysis has a significant impact on business decisions, as it allows companies to gain insights into customer opinions, monitor brand reputation, and make data-driven decisions.
Sentiment analysis can help businesses understand customer satisfaction levels, identify emerging trends, and tailor their marketing strategies accordingly.
Now, let’s delve into the key components of NLP for sentiment analysis.
Key Components of NLP for Sentiment Analysis
To effectively leverage NLP in AI for advanced sentiment analysis, it’s essential to understand the key components involved in this process.
NLP techniques play a crucial role in sentiment classification, allowing the system to accurately identify and categorize emotions expressed in text.
One important component is text preprocessing, which involves tasks such as tokenization, stemming, and removing stopwords. This step helps to standardize the text and eliminate noise that could affect sentiment analysis results.
Another key component is feature extraction, where relevant features are extracted from the text, such as n-grams, sentiment lexicons, and word embeddings. These features provide valuable insights for sentiment classification algorithms.
Finally, machine learning algorithms, such as Support Vector Machines (SVM) and Naive Bayes, are used to train the models and classify the sentiment of the text.
Understanding and effectively implementing these key components is crucial for accurate sentiment analysis using NLP techniques.
Techniques for NLP-based Sentiment Analysis
When conducting NLP-based sentiment analysis, it’s important to explore various techniques that can enhance the accuracy and effectiveness of the analysis. To achieve this, there are several NLP-based sentiment analysis techniques that have emerged due to advancements in NLP. These techniques include:
-
Lexicon-based approach: This technique involves using sentiment lexicons or dictionaries to assign sentiment scores to words or phrases in a text. These scores are then aggregated to determine the overall sentiment of the text.
-
Machine learning approach: This technique involves training machine learning models on labeled datasets to classify the sentiment of a given text. These models can learn patterns and features from the data to make accurate predictions on unseen texts.
-
Aspect-based sentiment analysis: This technique focuses on identifying and analyzing sentiments towards different aspects or entities mentioned in a text. It allows for a more granular analysis of sentiments.
Challenges in Implementing NLP for Sentiment Analysis
As we delve into the challenges of implementing NLP for sentiment analysis, it’s crucial to address the complexities that arise in utilizing these techniques effectively.
One of the primary implementation challenges is ensuring the accuracy of sentiment analysis results. NLP models heavily rely on training data, which must be diverse, representative, and labeled correctly. Obtaining such high-quality training data can be time-consuming and resource-intensive.
Additionally, sentiment analysis models often struggle with sarcasm, irony, and other forms of figurative language, leading to inaccurate results.
Another challenge is the need for continuous improvement of accuracy. NLP models require regular updates and retraining to adapt to evolving language patterns and incorporate new vocabulary. These ongoing efforts are necessary to maintain high accuracy levels and prevent models from becoming outdated.
Overcoming these implementation challenges and continuously improving accuracy are vital for effective sentiment analysis using NLP techniques.
Future Trends in NLP for Advanced Sentiment Analysis
In the future, we’ll witness an increased integration of NLP techniques with AI to enhance advanced sentiment analysis capabilities. This integration will bring about several exciting trends in the field of sentiment analysis. Here are three subtopics to explore:
-
Ethical implications:
As NLP becomes more sophisticated, questions around privacy, data protection, and algorithm bias will arise. Researchers and policymakers will need to address these concerns to ensure responsible and fair use of NLP in sentiment analysis. -
Beyond social media:
While social media platforms have been the primary focus for sentiment analysis, NLP can be applied to other domains such as customer reviews, market research, and political analysis. This expansion will allow businesses and organizations to gain valuable insights from a wide range of sources. -
Multilingual sentiment analysis:
NLP techniques will continue to advance in analyzing sentiment in multiple languages. This will enable businesses to understand customer sentiment in global markets and tailor their strategies accordingly.
These future trends in NLP for advanced sentiment analysis highlight the potential for further advancements in this field, with ethical considerations and expanded applications driving innovation.
Frequently Asked Questions
What Is the Significance of Sentiment Analysis in the Field of Artificial Intelligence?
Sentiment analysis plays a vital role in AI. It helps industries by providing insights into customer opinions, improving brand reputation, and enhancing customer experience. However, ethical considerations must be addressed when implementing AI for sentiment analysis.
How Does NLP Play a Crucial Role in Sentiment Analysis?
NLP plays a crucial role in sentiment analysis by leveraging its applications in advanced sentiment classification. Like a skilled detective, NLP algorithms analyze text, extract meaning, and categorize sentiments, empowering AI to understand human emotions more accurately.
What Are the Different Key Components of NLP That Are Utilized in Sentiment Analysis?
Key components of NLP utilized in sentiment analysis include lexical analysis, part-of-speech tagging, parsing, and machine learning techniques. These components enable accurate identification and understanding of sentiment expressions in text data.
Can You Explain Some Advanced Techniques Used in Nlp-Based Sentiment Analysis?
In advanced NLP-based sentiment analysis, we employ sophisticated techniques to enhance accuracy. Contextual embeddings, such as BERT, capture nuanced meanings by considering the surrounding words. These techniques enable more precise sentiment classification.
What Are the Major Challenges Faced in Implementing NLP for Sentiment Analysis and How Can They Be Overcome?
In implementing NLP for sentiment analysis, we face challenges such as overcoming limitations and handling sarcasm and irony. These obstacles can be overcome through techniques like contextual understanding and training models on diverse datasets.
Conclusion
In conclusion, leveraging NLP in AI for advanced sentiment analysis is a game-changer. The importance of NLP can’t be overstated as it enables us to accurately gauge people’s emotions and opinions. By understanding the key components and employing various NLP techniques, we can unravel the intricate nuances of sentiment analysis.
However, implementing NLP for sentiment analysis comes with challenges, such as language ambiguity and context comprehension. Nevertheless, future trends in NLP promise even more refined and accurate sentiment analysis capabilities.
Hanna is the Editor in Chief at AI Smasher and is deeply passionate about AI and technology journalism. With a computer science background and a talent for storytelling, she effectively communicates complex AI topics to a broad audience. Committed to high editorial standards, Hanna also mentors young tech journalists. Outside her role, she stays updated in the AI field by attending conferences and engaging in think tanks. Hanna is open to connections.
AI in Business
Unveiling the Power of Sentiment Analysis in AI for Business
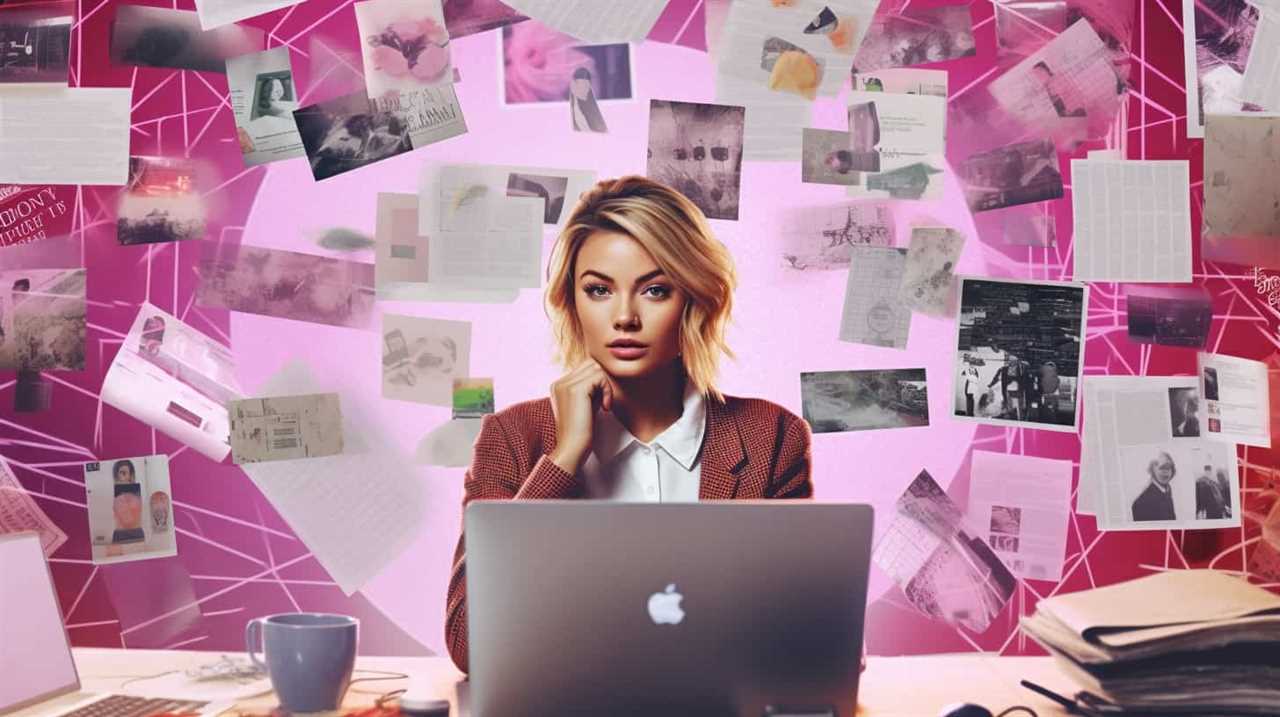
We made an exciting discovery: Sentiment analysis is revolutionizing AI for businesses.
Did you know that 85% of customers trust online reviews as much as personal recommendations?
In this article, we’ll delve into the power of sentiment analysis in the world of business AI. By harnessing the capabilities of natural language processing, sentiment analysis enables companies to analyze and interpret customer opinions and emotions.
Join us as we explore the benefits, techniques, applications, challenges, and future directions of sentiment analysis for business AI.
Key Takeaways
- Sentiment analysis helps businesses understand customer attitudes and opinions towards their products or services.
- Natural Language Processing (NLP) algorithms and machine learning models are used in sentiment analysis to analyze and interpret large amounts of text data.
- NLP enables businesses to efficiently process and understand social media sentiment, providing real-time monitoring and reliable insights.
- Sentiment analysis has real-world applications in monitoring public sentiment, improving customer satisfaction, and aiding in reputation management.
Overview of Sentiment Analysis in Business AI
In our article, we’ll provide a comprehensive overview of the role and significance of sentiment analysis in AI for businesses.
Sentiment analysis is a powerful technique that helps organizations extract valuable insights from customer feedback and online conversations. By analyzing sentiment, companies can understand customer opinions, emotions, and attitudes towards their products or services.
There are various sentiment analysis techniques available, including rule-based, machine learning, and hybrid approaches. These techniques involve the use of sentiment analysis tools, such as Natural Language Processing (NLP) algorithms, lexicons, and machine learning models. These tools help automate the process of sentiment analysis and can handle large volumes of data efficiently.
With sentiment analysis, businesses can make data-driven decisions, improve customer satisfaction, identify emerging trends, and enhance their overall brand reputation.
Benefits of Natural Language Processing in Sentiment Analysis
One significant benefit of Natural Language Processing (NLP) in sentiment analysis is its ability to accurately analyze and interpret large amounts of text data. NLP techniques enable businesses to efficiently process and understand social media sentiment, allowing them to gain valuable insights into public opinion and customer satisfaction.
Some benefits of NLP in sentiment analysis include:
-
Improved accuracy: NLP algorithms can identify and analyze sentiment with a high degree of precision and accuracy, providing businesses with reliable insights.
-
Efficient data processing: NLP enables businesses to process and analyze large volumes of text data in a relatively short amount of time, saving valuable resources.
-
Real-time monitoring: NLP techniques allow businesses to track social media sentiment in real-time, enabling them to respond promptly to customer feedback and concerns.
-
Enhanced decision-making: By leveraging NLP in sentiment analysis, businesses can make data-driven decisions based on a comprehensive understanding of customer sentiment and preferences.
Techniques for Training Sentiment Analysis Models
To effectively train sentiment analysis models, we employ various techniques that allow us to extract meaningful insights from large amounts of textual data.
One popular technique is transfer learning, which involves leveraging pre-trained models on vast datasets and fine-tuning them for specific sentiment analysis tasks. By using transfer learning, we can benefit from the knowledge and patterns learned by these models on general language understanding, saving time and resources.
Another technique is feature extraction, where we extract relevant features from the text that can help us classify sentiment. These features can include word frequencies, n-grams, or even more complex linguistic features like sentiment lexicons. By selecting and extracting the right features, we can enhance the accuracy and performance of our sentiment analysis models, making them more effective in understanding and classifying sentiment in textual data.
Real-world Applications of Sentiment Analysis in Business AI
Using transfer learning and feature extraction techniques, we can now explore real-world applications of sentiment analysis in business AI.
Sentiment analysis in social media allows companies to monitor and analyze public sentiment towards their brand, products, and services. By analyzing social media posts, comments, and reviews, businesses can gain valuable insights into customer opinions and identify trends and patterns.
Sentiment analysis in customer reviews helps companies understand customer satisfaction levels and identify areas for improvement. It enables companies to proactively address any negative feedback and improve customer experience.
Additionally, sentiment analysis can be used for reputation management, allowing businesses to track and manage their online reputation by identifying and addressing any negative sentiment.
Challenges and Future Directions in Sentiment Analysis for Business AI
As we delve into the challenges and future directions of sentiment analysis for business AI, we continue to explore its applications and the potential it holds for enhancing customer satisfaction and strategic decision-making.
Looking ahead, future advancements in sentiment analysis will likely focus on improving accuracy and reliability. This includes developing more sophisticated algorithms that can better understand complex emotions and subtle nuances in text. Additionally, advancements in natural language processing and machine learning techniques will enable sentiment analysis systems to become more context-aware, taking into account factors such as sarcasm and irony.
However, with these advancements also come ethical implications. As sentiment analysis becomes more powerful and pervasive, there’s a need to address issues of privacy, bias, and the responsible use of customer data. Striking the right balance between innovation and ethical considerations will be crucial for the future of sentiment analysis in business AI.
Frequently Asked Questions
Can Sentiment Analysis Be Used in Industries Other Than Business Ai?
Sentiment analysis can indeed be applied in industries beyond business AI. For instance, sentiment analysis finds use in healthcare by analyzing patient feedback. Furthermore, it is employed in political campaigns to gauge public opinion and tailor messaging accordingly.
What Are the Limitations of Using Natural Language Processing in Sentiment Analysis?
Sentiment analysis accuracy and challenges in natural language processing include bias, sarcasm, and context understanding. These limitations hinder the ability to accurately interpret emotions, making sentiment analysis a complex task in AI for business.
How Long Does It Typically Take to Train a Sentiment Analysis Model?
Training time for sentiment analysis models varies depending on the dataset size and complexity. Generally, it takes a few hours to a few days. However, longer training times can lead to higher model accuracy, ensuring reliable sentiment analysis results.
Are There Any Ethical Concerns Surrounding the Use of Sentiment Analysis in Business Ai?
There are ethical implications and privacy concerns surrounding the use of sentiment analysis in business AI. We must consider the potential misuse of personal data and the potential for biased or discriminatory outcomes.
What Are the Potential Future Advancements and Developments in Sentiment Analysis for Business Ai?
In the future, we can expect exciting advancements and developments in sentiment analysis for business AI. AI technologies will continue to evolve, enabling more accurate and nuanced analysis of sentiment, leading to better decision-making and customer insights.
Conclusion
In conclusion, sentiment analysis holds immense potential in shaping the future of AI for business. By harnessing the power of natural language processing techniques, organizations can gain valuable insights into customer sentiments, enabling them to make data-driven decisions.
However, like any evolving technology, sentiment analysis faces challenges and requires continual advancements. As the field progresses, it’s crucial for researchers and practitioners to address these challenges and explore new avenues to further enhance the accuracy and applicability of sentiment analysis in the realm of business AI.
Hanna is the Editor in Chief at AI Smasher and is deeply passionate about AI and technology journalism. With a computer science background and a talent for storytelling, she effectively communicates complex AI topics to a broad audience. Committed to high editorial standards, Hanna also mentors young tech journalists. Outside her role, she stays updated in the AI field by attending conferences and engaging in think tanks. Hanna is open to connections.
AI in Business
Why Natural Language Processing Is a Game-Changer for Customer Experience
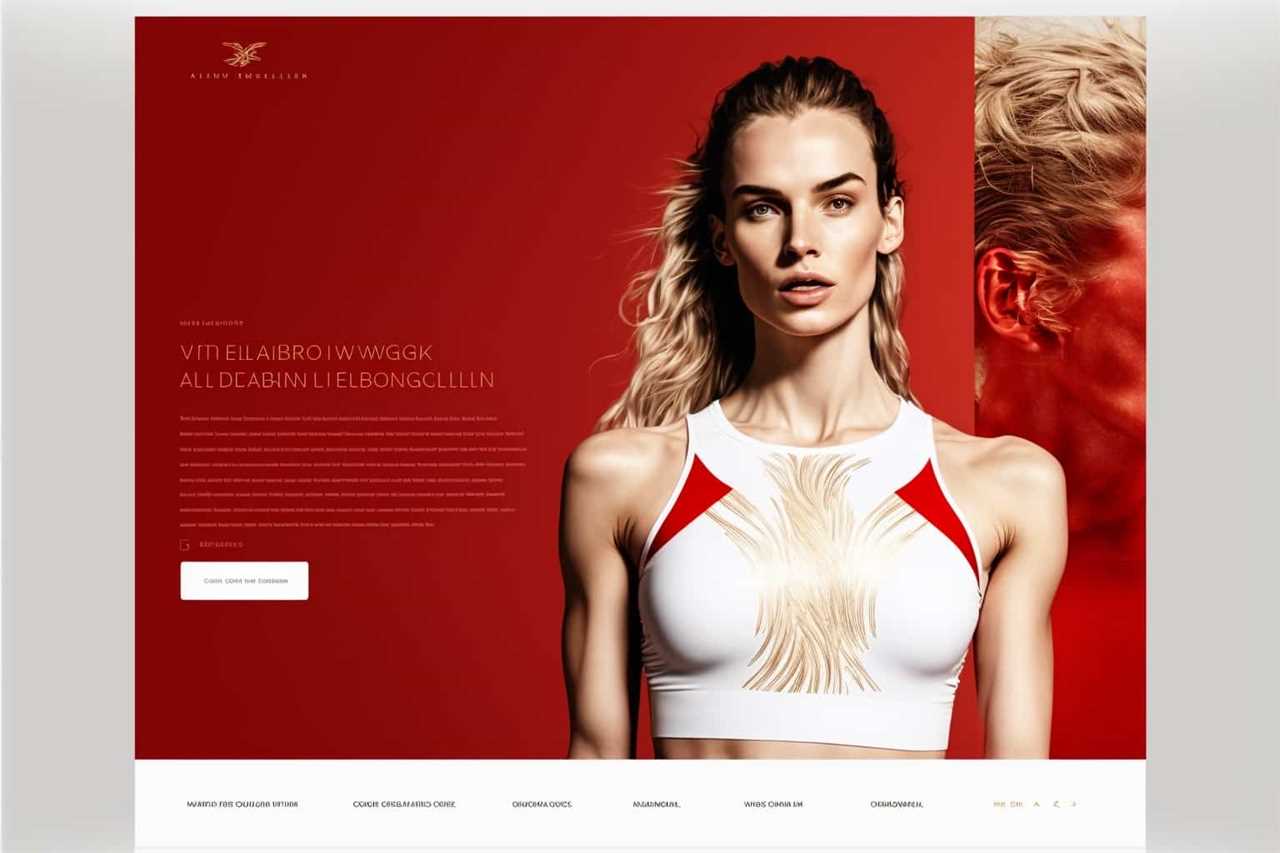
Imagine a situation where customer interactions are improved, understanding of customer needs is increased, and customer support becomes more effective. Enter the world of Natural Language Processing (NLP).
With NLP technology, we can transform customer experiences into personalized journeys. Through the power of language processing, we can unlock a new level of customer satisfaction.
In this article, we will explore why NLP is revolutionizing the way businesses connect with their customers. Get ready to embark on a journey towards mastery in customer experience.
Key Takeaways
- AI chatbots revolutionize customer service by providing automated support.
- Natural language processing enables accurate interpretation of customer intents.
- NLP technology helps businesses enhance their services according to customer needs.
- NLP technology contributes to a positive customer experience.
Enhanced Customer Interactions Through AI
We have witnessed a significant improvement in customer interactions through the implementation of AI technologies.
AI powered chatbots have revolutionized the way businesses handle customer queries and concerns. These chatbots provide automated customer service, allowing businesses to respond to customer inquiries promptly and efficiently.
By leveraging advanced natural language processing techniques, AI chatbots can understand and interpret customer intents accurately, providing personalized and relevant responses. Additionally, sentiment analysis is used for feedback analysis, enabling businesses to gauge customer satisfaction levels and identify areas for improvement.
This valuable insight helps companies tailor their products and services to meet customer needs effectively. Furthermore, AI technologies enable proactive customer engagement, allowing businesses to anticipate customer needs and provide proactive support.
Improved Understanding of Customer Needs
By implementing natural language processing, businesses can gain a deeper understanding of their customers’ needs. This enhanced customer insights allows companies to optimize their product recommendations and deliver a more personalized experience. Natural language processing enables businesses to analyze large volumes of customer data, such as reviews, feedback, and social media interactions, to identify patterns and trends. This deeper understanding of customer needs enables businesses to tailor their products and services to meet specific demands. Through the use of advanced algorithms, businesses can generate optimized product recommendations, increasing the likelihood of customer satisfaction and loyalty. The following table illustrates how natural language processing can enhance customer insights and optimize product recommendations:
Enhanced Customer Insights | Optimized Product Recommendations | Improved Customer Experience |
---|---|---|
Analyzing customer data | Generating personalized suggestions | Meeting specific demands |
Identifying patterns and trends | Increasing customer satisfaction | Enhancing customer loyalty |
Tailoring products and services | Improving customer retention | Delivering personalized experiences |
Streamlined Customer Support With NLP
Implementing natural language processing (NLP) in customer support offers several benefits. One key advantage is the use of an automated ticketing system, which categorizes and routes customer inquiries to the appropriate department or agent. This reduces manual effort and response times, improving efficiency and ensuring faster and more accurate support for customers.
Another advantage of NLP is the integration of chatbots. These virtual assistants can understand and respond to customer queries in a conversational manner. Equipped with NLP capabilities, chatbots provide instant assistance, answer frequently asked questions, and handle basic tasks. This frees up human agents to focus on more complex customer issues.
Personalized Customer Experiences With Language Processing
One of the key benefits of language processing is its ability to provide personalized customer experiences. By analyzing customer data and understanding their preferences, businesses can tailor their marketing strategies to individual customers.
Language processing allows companies to gather valuable insights from social media platforms, enabling them to understand customer sentiment and engage with their audience effectively. With the help of language processing in social media, businesses can identify customer needs, interests, and buying behaviors, allowing them to deliver targeted and personalized marketing messages.
This not only enhances the customer experience but also increases the likelihood of conversion and customer loyalty. By utilizing language processing technologies, businesses can create personalized marketing strategies that resonate with their customers, ultimately leading to increased customer satisfaction with NLP technology.
Increased Customer Satisfaction With NLP Technology
With the implementation of natural language processing technology, we can significantly enhance customer satisfaction.
NLP applications in sales and marketing have revolutionized the way businesses interact with their customers. By analyzing and understanding customer feedback, NLP technology helps companies identify pain points and areas for improvement. This enables them to offer personalized solutions and address customer concerns more effectively.
In addition, NLP’s impact on customer loyalty can’t be underestimated. By providing real-time support and personalized recommendations, NLP technology builds trust and strengthens the customer-business relationship. Customers feel valued and understood, leading to increased satisfaction and loyalty.
Furthermore, NLP technology allows businesses to gather valuable insights from customer conversations, which can be used to improve products, services, and overall customer experience.
Frequently Asked Questions
How Does Natural Language Processing Enhance Customer Interactions Through Ai?
Natural language processing improves chatbots and enhances sentiment analysis, allowing us to better understand and respond to customer interactions. By leveraging AI, it revolutionizes customer experience by providing personalized and efficient communication.
How Does NLP Technology Improve Understanding of Customer Needs?
Improving customer interactions and enhancing customer feedback analysis, NLP technology enables us to better understand customer needs. It helps us analyze and interpret their language, leading to more personalized and effective customer experiences.
What Are the Benefits of Streamlined Customer Support With Nlp?
Efficiency and automation are the benefits of streamlined customer support with NLP. It helps us provide faster and more accurate responses, improving customer satisfaction and allowing us to handle a higher volume of inquiries.
How Does Language Processing Enable Personalized Customer Experiences?
Language processing enables personalized customer experiences by adopting a customer centric approach and providing personalized recommendations. By understanding and analyzing customer data, NLP allows us to tailor interactions and offer relevant solutions, enhancing overall satisfaction.
What Are the Ways in Which NLP Technology Increases Customer Satisfaction?
Improved communication and enhanced problem solving are some ways in which NLP technology increases customer satisfaction. It allows us to understand and respond to customer needs more effectively, leading to a more personalized and efficient customer experience.
Conclusion
In conclusion, natural language processing is a total game-changer for customer experience. With AI-enhanced interactions, businesses can better understand customer needs and provide streamlined support.
This technology allows for personalized experiences and ultimately leads to increased customer satisfaction.
So, if you want your customers to be as happy as a kid in a candy store, incorporating NLP into your customer service strategy is a no-brainer. Trust me, they’ll be singing your praises like a choir of angels.
Hanna is the Editor in Chief at AI Smasher and is deeply passionate about AI and technology journalism. With a computer science background and a talent for storytelling, she effectively communicates complex AI topics to a broad audience. Committed to high editorial standards, Hanna also mentors young tech journalists. Outside her role, she stays updated in the AI field by attending conferences and engaging in think tanks. Hanna is open to connections.
AI in Business
Humane’s AI Pin: A Closer Look at the Revolutionary Wearable
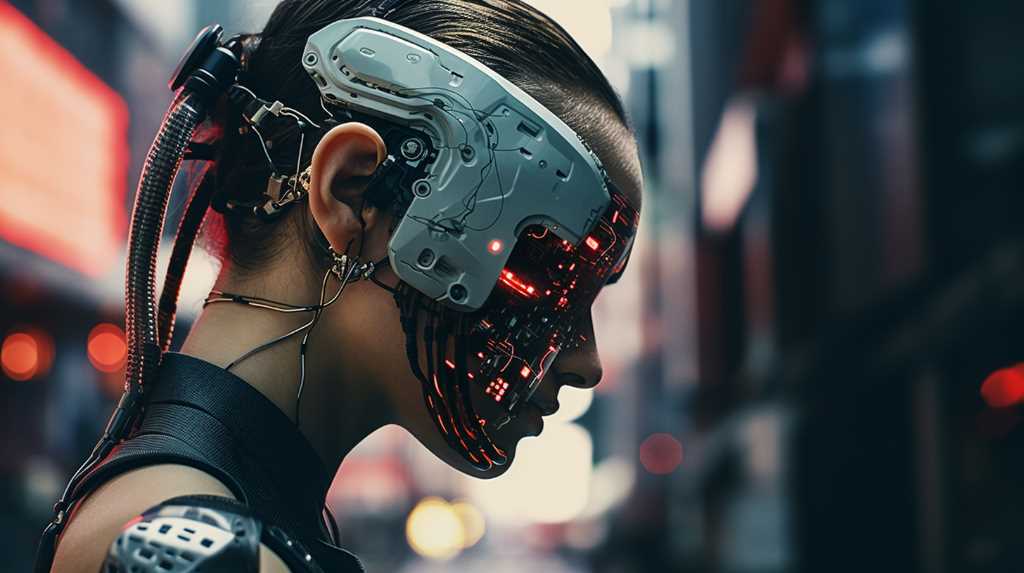
Time Magazine Recognizes AI Pin as a Top Invention of 2023
Humane, the innovative technology company, is gearing up for the launch of its highly anticipated AI Pin on November 9th. To much surprise, the device has already been honored as one of Time Magazine’s “Best Inventions of 2023,” alongside other cutting-edge gadgets such as the Framework Laptop 16 and the Samsung Galaxy Z Flip 5. This recognition has heightened the excitement surrounding the AI Pin, providing us with a more detailed look at this revolutionary wearable.
The AI Pin: A Fusion of Style and Intelligent Technology
While details have been scarce, the latest write-up provides some intriguing insights into the AI Pin. The device is designed to attach magnetically to your clothing, offering a seamless integration into your everyday life. Powered by a combination of proprietary software and OpenAI’s GPT-4, the AI Pin boasts a range of impressive features.
Trust Light: Ensuring Privacy and Transparency
One standout feature of the AI Pin is its “Trust Light.” This illuminated indicator activates whenever the device’s camera, microphone, or other sensors are recording data. This transparency aims to address concerns about privacy and data collection, setting the AI Pin apart from its competitors.
A New Kind of Wearable: The Promise of the AI Pin
Humane has been building anticipation for the AI Pin for months, starting with a captivating TED talk by co-founder Imran Chaudhri in April. Chaudhri described the AI Pin as a “new kind of wearable device and platform,” emphasizing that it operates independently of smartphones or other devices. This bold claim has left many intrigued about the inner workings and functionality of the AI Pin.
A Race to Revolutionize AI-Powered Gadgets
While Humane has generated significant buzz around its upcoming release, it faces fierce competition in the AI gadget market. Other companies, such as Rewind and a collaboration between Sam Altman and Jony Ive, are also vying to create innovative devices that harness the power of artificial intelligence. Additionally, the rapid growth of smart glasses with AI assistants, spearheaded by Meta and Amazon, has contributed to an industry-wide push to explore the potential of AI-powered hardware.
Unveiling the Future: Launching on November 9th
Despite the lingering questions surrounding its functionality, the AI Pin remains on track for its highly anticipated launch on November 9th. As the unveiling date approaches, we eagerly await a deeper understanding of what the AI Pin can do and how it will enhance our daily lives. Will this be the wearable of the future? Only time will tell.
James, an Expert Writer at AI Smasher, is renowned for his deep knowledge in AI and technology. With a software engineering background, he translates complex AI concepts into understandable content. Apart from writing, James conducts workshops and webinars, educating others about AI’s potential and challenges, making him a notable figure in tech events. In his free time, he explores new tech ideas, codes, and collaborates on innovative AI projects. James welcomes inquiries.
-
AI News4 weeks ago
The Role of AI in Disaster Preparedness and Emergency Response Education
-
AI News2 weeks ago
AI-Driven Personalization in E-commerce: Enhancing Customer Experience
-
AI News3 weeks ago
AI in Archaeology: Uncovering History With Advanced Technology
-
AI News2 weeks ago
AI in Renewable Energy: Advancing Green Technology Education and Implementation
-
AI News4 weeks ago
AI-Powered Energy Management: Sustainable Solutions for Businesses and Schools
-
AI News4 weeks ago
AI in Library Sciences: Transforming Information Management and Access
-
AI News4 weeks ago
AI in Fashion: Revolutionizing Design, Production, and Retail
-
AI News3 weeks ago
The Ethics of AI in Criminal Justice: Balancing Fairness and Public Safety